By: Madipally Sunil Veer Kumar
In today's ever-changing technology landscape, it is imperative that systems remain reliable and perform optimally. To achieve this, it has become vital to monitor and understand the internal state of systems, a practice known as observability. Artificial intelligence (AI) has revolutionized observability by enabling proactive issue identification and resolution, as well as predicting future infrastructure usage for effective resource scheduling, thereby avoiding overprovisioning.
AI Advantage:
Observability is greatly enhanced through real-time analysis of vast amounts of data using machine learning algorithms, a task that is not feasible for humans.
Machine learning algorithms used in observability should possess a distinctive feature: the ability to overlay trace data captured with observability tools onto these algorithms. This approach drastically reduces training time, as the directionality of impact metrics is already identified as part of the trace, thus significantly reducing training costs. Other machine learning systems that do not adopt this method often incur high training times and costs.
Anomaly Detection:
Anomaly detection involves identifying events that deviate significantly from standard behaviors or patterns. Anomalies can manifest as outliers, novelties, or exceptions.
When an abnormality is detected, customers receive alerts, which is particularly beneficial for those who may not be aware of their service level agreements (SLAs) or are new to the observability journey. It is crucial for customers to select an observability solution that includes this feature, as it detects anomalies not only based on single metrics but also across multiple metrics.
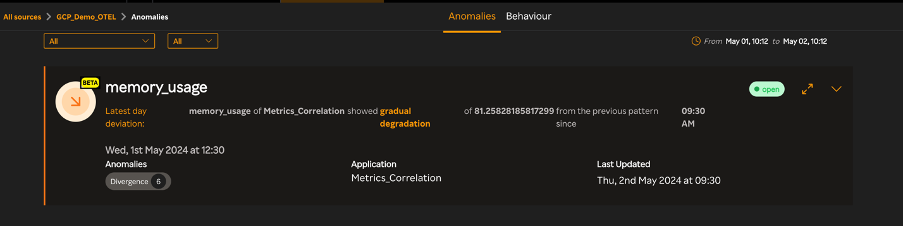
Predictive Analytics:
The purpose of predictive analytics is to forecast future events based on current and historical data. In observability, the objective is to predict system performance, anomalies, and scaling, thereby assisting organizations and SREs in better preparation and avoidance of future challenges and issues. This can lead to cost reduction by forecasting the required RPMs and system resources, thereby avoiding over-provisioning.
Organizations should prioritize observability tools that not only forecast based on historical data but also learn and incorporate seasonal patterns. This is crucial, as failing to consider seasonality can lead to inaccurate forecasting, eroding customer confidence in the tool.
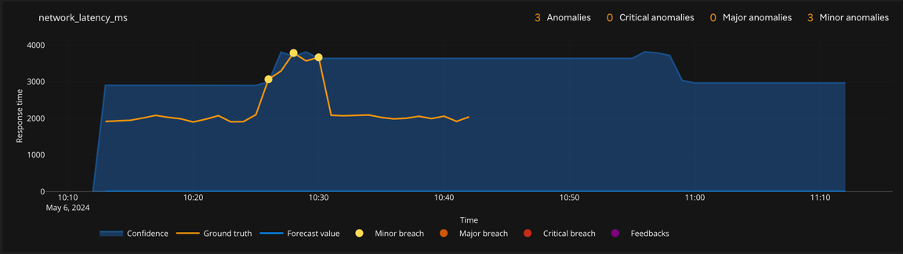
Causation & Correlation:
This process aims to identify the Root Cause Analysis (RCA) by correlating data across different metrics and sources to generate an impact analysis. This analysis helps determine which metric changes will affect others, essentially identifying the blast radius based on past data and patterns. By continuously monitoring for these patterns in current data, it becomes possible to identify and inform SREs about potential RCAs and solutions.
This feature is crucial, yet many observability tools lack it. It is essential for organizations to choose tools that include this functionality, as it can significantly reduce SRE workloads and improve preparedness by anticipating and avoiding issues before they occur.
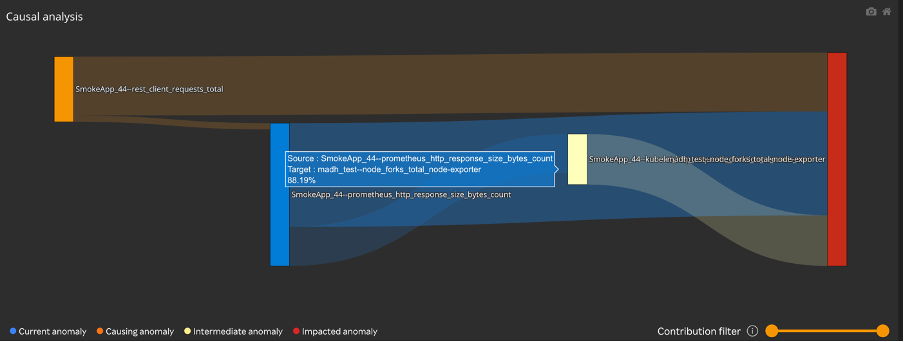
Future Trends:
Speculate on the future of AI in observability, including emerging technologies and trends shaping the landscape. Discuss the potential impact of advancements such as reinforcement learning, federated learning, and autonomous systems on the evolution of observability practices.
Conclusion:
Summarize the transformative potential of AI in Observability, including emerging technologies and trends shaping the Observability landscape. While there are multiple areas where AI can contribute to Observability, only some of them are listed above.
It is important for organizations to evaluate tools that not only perform anomaly detection, prediction, and causation & correlation, but also have machine learning algorithms that can remember seasonality for better and more accurate predictions. Additionally, algorithms that can overlay trace/topology data to reduce training times, as well as identify blast radius and produce causation graphs, are crucial. This allows SREs to be better prepared and avoid issues before they happen.
At Rakuten SixthSense, we have trained our AI to perform all the tasks specified above, making us the only tool in the market that offers comprehensive end-to-end AI integration of observability tools.
Call to Action:
Encourage readers to explore AI-powered observability solutions as specially Rakuten SixthSense and embrace innovation in monitoring and optimization strategies to stay ahead in today’s dynamic IT landscape.