By: Rahul Raj
Use Case
2024 is a year of GEN AI and LLM. Today, everyone is talking about building their own GEN AI solutions and using them for their business needs. But what about the platform or infrastructure? Nobody wants to manually deploy tens or hundreds of models by logging into machines manually. How can we automate this process and make it self-serve? This article discusses the same topic.
Hybrid Approach
One of the must-have requirements for any AI/ML model is a GPU, and in some cases, it's a TPU depending on the complexity of the model solutions. Usually, any multinational organization provides GPU/TPU infrastructure by leveraging either the famous cloud providers like GCP, Azure, AWS, or using their own data centers. The most optimized and better solution is to have an architectural design that can leverage the power of all clouds as well as on-premises solutions. High availability, scaling, and resource optimization can be achieved by leveraging the flexibility of a containerized environment, which is widely accepted and used throughout the industry.
Architecture Design
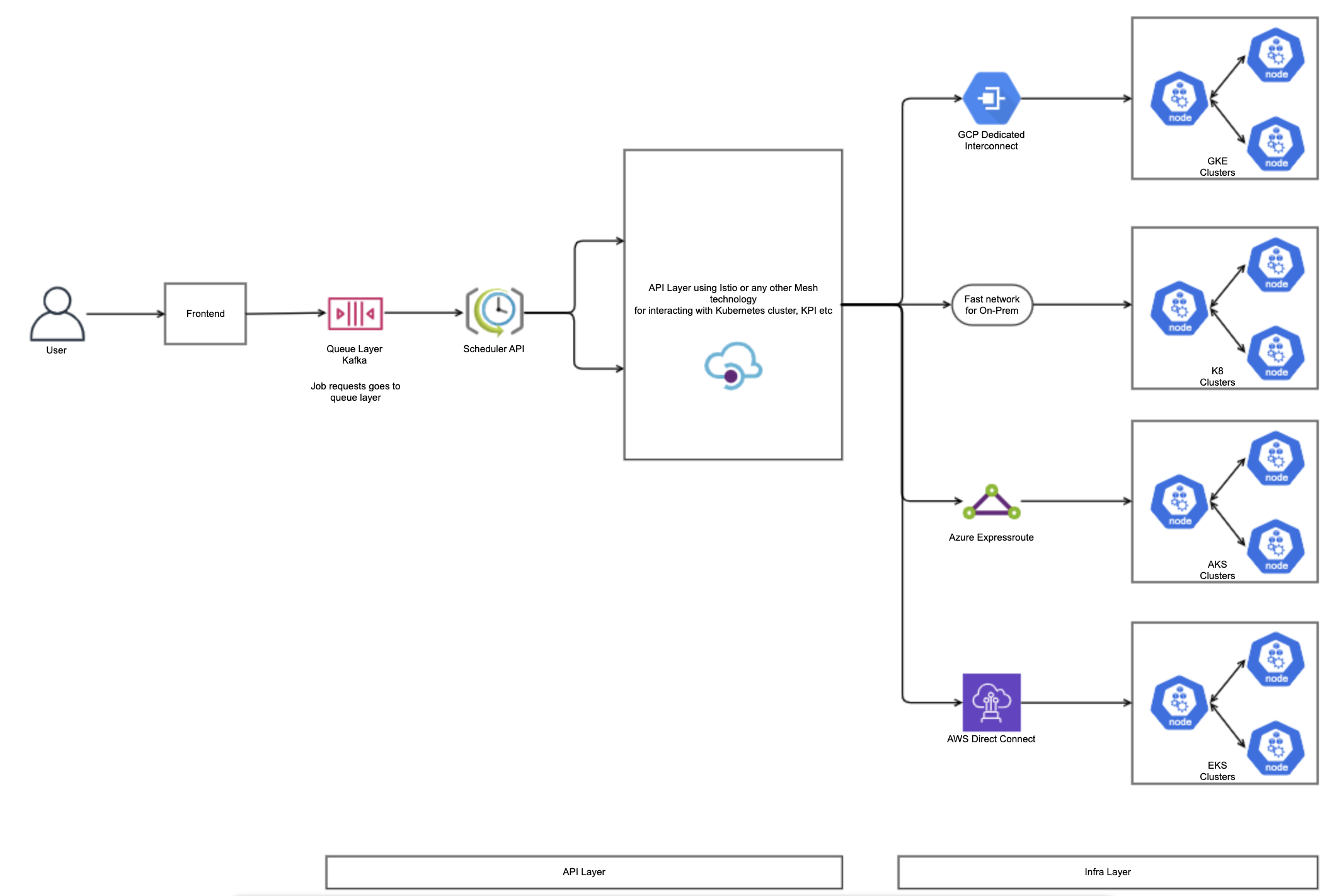
This is the conceptual engineering architecture design which focuses on utilizing the power of GKE, AKS, EKS, and on-prem K8s built using Kubeadm's. In this architecture, the following are the major components:
- Queue & Scheduler Layer: The Queue & Scheduler layer can be built using Kafka & Scheduler API to prioritize job requests from users based on high/medium/low priority.
- Compute API Layer: The Compute API layer has a major role to play here. It has the following functionalities:
- Model Deployment: The API layer should be able to deploy the models on K8 clusters in the form of K8 deployments or K8 jobs.
- Model Serving: It should be able to leverage the K8 features of service and ingress layer and expose the model for interactive usage.
- Resource Management: It should be able to trace the required resource availability across different cloud clusters using Resource Quotas and deploy the model to the correct infra for optimum resource management.
- Continuous Model Training: It should be able to track and deploy the long-running streaming models by leveraging cron jobs or other scheduling features of K8s.
- Resource Reservation: Taints & Tolerations K8 features provide the facility to reserve dedicated resources for jobs. It can be used in conjunction with K8 labels to reserve dedicated resources for dedicated jobs.
- Hybrid Network Connections: Different cloud providers provide their specific tools for connection to on-prem environments. For example, GCP provides dedicated interconnect, Azure provides ExpressRoute, and similarly, AWS provides AWS Direct. Using these tools, connections can be established with on-prem environments.
Conclusion
From our experience, Kubernetes combined with different cloud providers has proven to be an effective solution for large-scale model deployments and serving.
I hope you found this blog useful.
Thank you.
References