By: Sayantan Chattopadhyay
Researchers have found that the costs associated with acquiring new customers can be five times (or more) higher than those associated with maintaining relationships with existing customers. Thus, once acquired, businesses should use customer relationship management (CRM) to nurture customer relationship to prevent them from churning. In this blog, I have explored the user aspects of customer interaction with an ecommerce business and how we could use it for Churn prediction and eventually prevention so that we are able to improve the customer experience and create a win-win situation for both marketer and the customer.
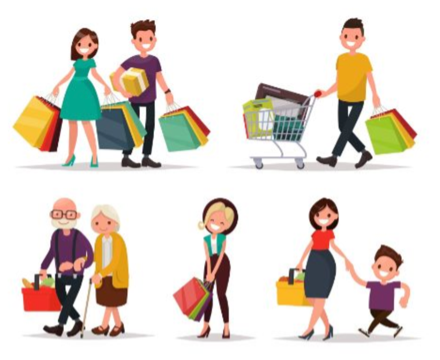
Customer Churn Prediction
AI based customer churn prediction techniques can be used to identify customers who are likely to stop using the product or service offerings of a firm. Churners could be of two types: intermittent loss (these customers are likely to return after some time) and permanent loss. AI models can predict the probability of a customer churning in a certain period of time, generally one to three months. Then, marketing strategies or interventions can be used to prevent churn.
What are the frameworks that could be used for churn prediction?
Following are the different types available:
- Recency and Frequency (RF) based Statistical Model: Pareto/NBD and BG/NBD models assume that while active, # transactions made by a customer follows Poisson distribution also the time between transactions is distributed exponential with transaction rate λ. The BG/NBD model is easier to implement and converges much faster than Pareto/NBD. Unsupervised statistical models don’t need feature variables like demographic details etc and thus could be used if customer information is limited.
- Binary Classification Model using Static Features: The customer churn problem is widely seen as a binary classification problem and solved by supervised machine learning algorithms, such as decision tree , association analysis, logistics regression, support vector machine (SVM) and artificial neural network (ANN). This model is widely used and has low time complexity.
- Recency, Frequency and Monetary (RFM) based Sequence Model: Results show that RFM variables in combination with LSTM neural networks or other sequence model have larger top- decile lift and expected maximum profit metrics than regularized binary classification models with commonly-used demographic features.
Customer Churn Prevention
Traditionally, marketers intervene to prevent customer churn by either providing discounts or other offers to the customer or do extensive market research to hear the voice of the customer and make necessary changes to the product or service offerings based on the customer’s ever changing need and want. AI based models could help the marketer decide the following:
- Why Customers are churning?
- Which segment of customers to prevent from churning?
- How to prevent high priority customers from churning?
- When to intervene to prevent customers from churning?
Why are customers churning?
The reason for customer churn could often be sublimal or driven by market competition. However the top five or ten features from the churn prediction model could suggest following:
- Demographic Details of Churners: which segment of users are likely to churn.
- User Activity: how engaged are the churning users
- Behaviour and Interest: what kind of products or services are churners interested in
How segment of customers to prevent from churning?
Let us assume that there are three users Sam, John and Rocky who use an e-commerce app to buy products online. By predicting the lifetime value and churn probability, we could cluster them into three category of users. Sam is a high risk, as churn probability in the next three months is high (more than 0.75), and high lifetime value user while John is a high risk and low lifetime value user. In order to retain Sam or John, if the firm plans retention spend, in the form of discount coupon or offers, then retention of Sam would be of higher priority than John. At the same time, the firm needs to nurture Rocky with retention spend to proactively reduce the likelihood of churning in future.
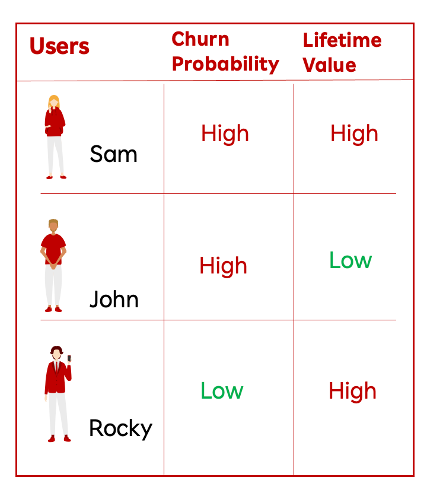
How to prevent customers from churning?
Here are some of the methods that could be used to prevent churn:
- Recommendation: Recommender systems primarily aim to reduce the user’s effort and time
required for searching relevant information over the internet. Good recommendations create
a feeling of serendipity in customers and improve user experience. Both personalized and non-
personalized recommendations could be used to engage users. Following are some SOTA
models that could be used:
Non-personalized - Capturing Popularity Trends: A Simplistic Non-Personalized Approach for Enhanced Item Recommendation
Personalized - Multi-Task Recommendations with Reinforcement Learning - Counterfactual Analysis: Counterfactual analysis could be done using the churn prediction model mentioned above on the top five or ten actionable features. There are models like Diverse Counterfactual Explanations for Machine Learning Classifiers (DICE) which could be used to generate counterfactuals and determine what changes need to be made to convert a churner into a non-churner.
Following is an example of counterfactual analysis where we find that Sam and John are currently not using mobile app (Use of Mobile App feature value is 0). If we predict the churn probability by setting this feature value to 1, we find that use of mobile app by Sam could potentially decrease her churn probability from 78% to 48%. Thus marketer would have to intervene and recommend the use of mobile app to Sam by using a campaign. It is worth noting that Time Spent Browsing feature value could be increased if recommendations are given which lead to enhanced user engagement.
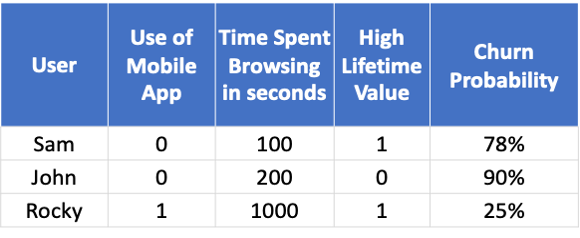
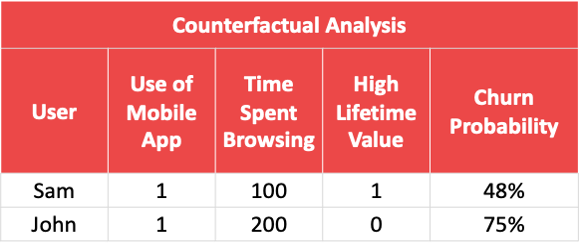
When to intervene to prevent customers from churning?
To determine the right time to intervene, sequence models could be used to model sequence of historical activity and predict the churn probability. Once the churn probability crosses a threshold (for example 0.75), discount or other offers could be provided to the user. Following is a schema of how marketing intervention could be planned by an online subscription based service firm:
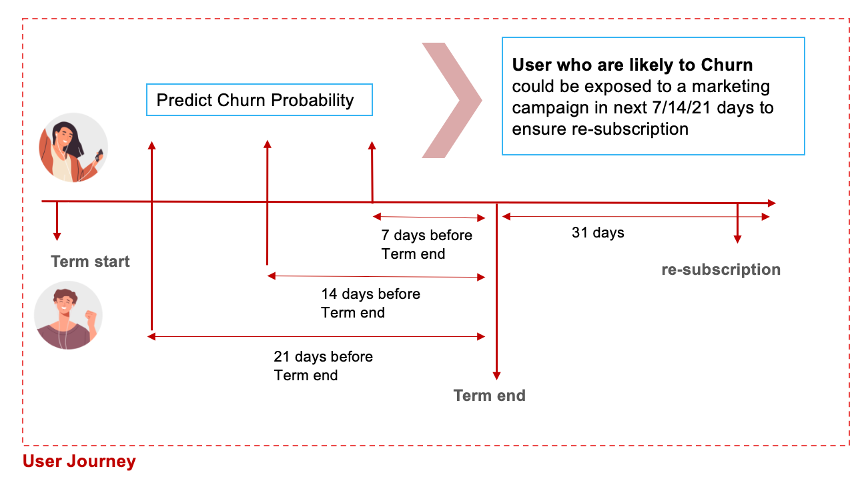
Conclusion
AI models are being widely used in increasingly competitive markets, which are often characterized as red oceans. In order to better their product and service offerings, businesses need to learn more about customers and their changing needs and wants, ethical use of AI could help businesses improve the lives of their customers.
References
- Li, Xueling, and Zhen Li. "A Hybrid Prediction Model for E-Commerce Customer Churn Based on Logistic Regression and Extreme Gradient Boosting Algorithm." Ingénierie des Systèmes d'Information 24.5 (2019).
- Mena, C. Gary, et al. "Churn prediction with sequential data and deep neural networks. a comparative analysis." arXiv preprint arXiv:1909.11114 (2019).
- Roy, D., Dutta, M. A systematic review and research perspective on recommender systems. J Big Data 9, 59 (2022). https://doi.org/10.1186/s40537-022-00592-5
- https://www.blueoceanstrategy.com/blog/red-ocean-strategy/