By: Ashish Nanjiani
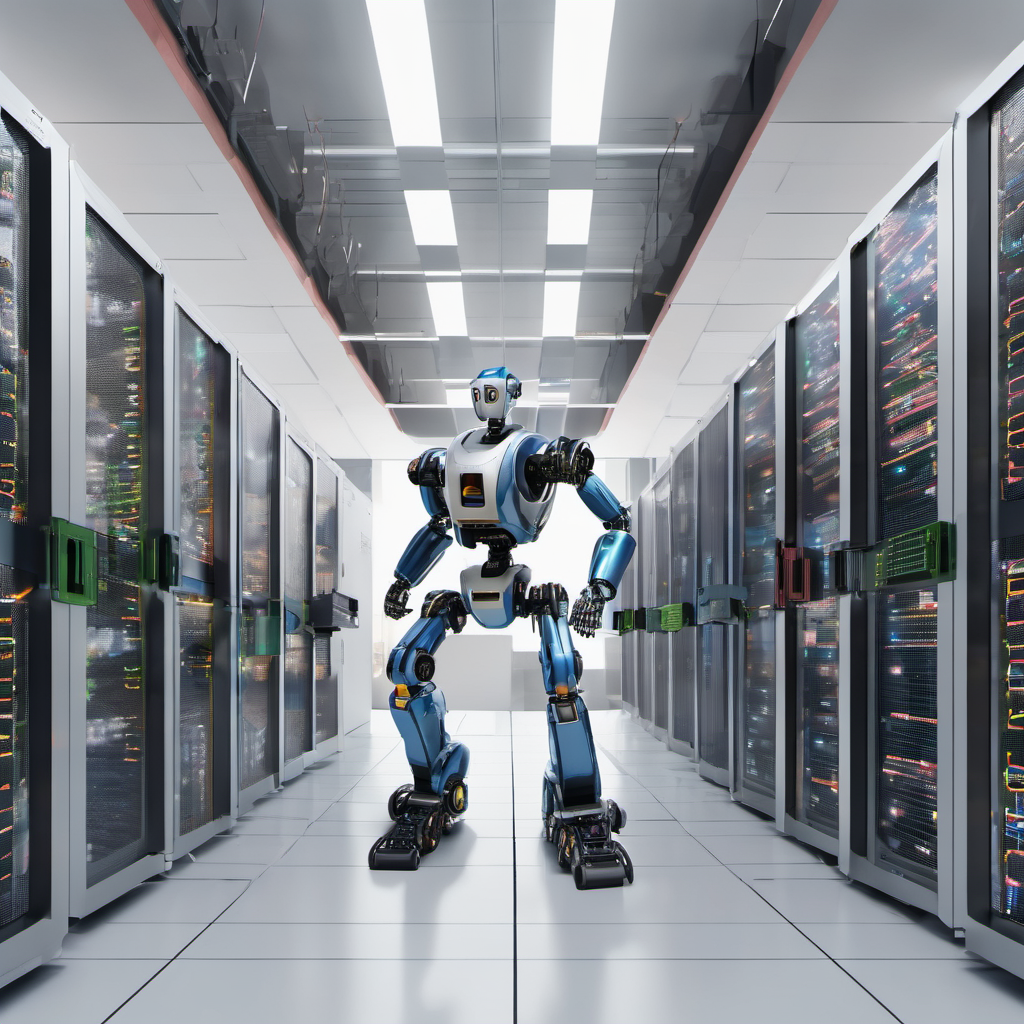
AI seems to be taking over the world right now. The pace of innovation with AI is surpassing any other innovation in the history of mankind. It is almost like a genie out of the bottle and is fulfilling the wishes of many. So, if you are an IT infrastructure person, I am sure your boss would have called you up by now and asked one of these questions: ‘What are we doing about AI?’ ‘How can we use AI to solve our problems?’ ‘How can AI be used in infrastructure?’ ‘Everyone is talking about GenAI, why are we not doing anything about it?’ ‘When can we roll out Ainization program in our department?’ So, if you are currently doing tons of Google searches or asking GenAI to give you ideas, here’s a blog that can help you save some time. While the field itself is evolving at a rapid pace, this article will give you some clues that you can use to investigate further.
Observability: The world of infrastructure is complex; we deal with thousands of machines all the way from network devices to computing. We also have many applications, like databases, running on top of underlying hardware. All these systems generate tons of logs and alerts. While the scale may differ from one company to another, the tech stack looks pretty much the same. Interestingly, irrespective of which company you work for or what title you may have, the most important job for any infrastructure professional is to keep the infrastructure up and running! Downtime is the worst nightmare come true!! It is the only thing that keeps us awake at night. And while we have many observability tools to choose from, they are all limited by their functionality today. Imagine having a tsunami warning system that gives you just enough time to save as many lives as possible. While preventing a tsunami is not in your hands, and neither is the failure of hardware, if you can get enough warning/time to prevent the disaster, you will have the genie you are looking for! While all the observability tool vendors promise they have nailed it, we all know the truth about what happens when the rubber meets the road. In my 25+ years of IT experience, I have personally evaluated many such tools, but all have failed to meet my expectations. However, with the advancement of AI, things seem to be changing. Given the maturity of ML models to scale and ingest huge amounts of machine log, metrics, and alert data, these models can be trained to not only detect anomalies but also predict failures or forecast capacity issues that can lead to outages. Difficult issues, like application performance issues, can be quickly understood by using AI. While it is still early, true AIOPS capabilities seem to be just around the corner.
Data centre optimization: With thousands of machines running in a data center, a few problems that continue to haunt any data center manager are how to optimize energy spending and how to avoid waste. Given the amount of investment required to operate/manage a DC, it is an ongoing battle to have the data center run at optimal cost/performance. AI can come to the rescue. With AI, one can optimize the cooling needs within the DC. AI models (with the help of IoT devices installed inside the DC) can control the amount of cooling required in a particular rack, optimize the airflow, control the vents, regulate the power requirements, and ensure there is no waste. This can significantly reduce the overall electricity cost of operating a DC. Not only that, but an AI-based system can also detect issues quickly and initiate a fix before the issue results in an outage. We also know that in any data center, there is a lot of waste in terms of CPU/memory/storage. With the thousands of machines running, how can we optimize the usage so that we can bring the cost of operations down? AI can again help to not only detect waste but also recommend and act. Many FINOPS tools currently available on the market are now exploring AI capabilities to accurately determine the utilization of hardware and intelligently move workloads to reduce waste.
Customer Support: This is one of the areas that has been disrupted by GenAI-based tools. Given that this field is heavily dependent on knowledge base/documentation, it is a perfect area where LLMs can help customers get answers to the most common issues. While we have seen several advancements in chatbots, a GenAI-based chatbot using LLM, Langchains, can provide the most accurate answer to a customer query. Taking a step ahead, not only can this help answer queries, but many queries can be converted into actions. For example, let's say a customer runs a query on how to provision a database on a particular cloud. The customer answers a few prompts, and the chatbot replies with a process on how to deploy a database and which database is the best to provision at an optimal price and performance. The customer is happy with the reply and now clicks a button to deploy. The chatbot then connects to either the underlying runbooks or Terraform, generates the required code, and completes the provisioning. Sweet!
Security: The third area where AI can be of tremendous help is in the field of security. As we know, when it comes to security, every second counts. With bad actors continuously trying to break through your systems, it’s almost impossible for humans to monitor all devices 24x7. While many systems exist today that can monitor your traffic, having an AI-based threat management system can take it to the next level. AI can analyze data for unusual patterns and behaviors, which can help detect threats early. AI-powered systems can automate threat detection processes, providing real-time monitoring and rapid response times. After all, AI does not need a chai break.
Team productivity: In addition to the above, using AI can improve the productivity of your team members. For example, implementing a GenAI-based bot on top of your databases can simplify querying by allowing natural language input, thus avoiding the need to insert large SQL statements that can be error-prone. Code bots can assist in improving existing code, writing new code, upgrading current code, or even creating test cases. New tools enable product managers to generate wireframes with just a few prompts, and they can assist project managers in creating project plans or Gantt charts.
These are just a few examples of how AI can assist infrastructure professionals. There are many other opportunities where AI can come to the rescue. The key is getting your team ready. Is your team skilled enough to take advantage of what AI offers? Do they understand how AI works? Most of us think we need data scientists and programmers to build models and AI applications, but that’s not true. There are many open-source and vendor-based AI models that can be used without the need for any coding. So instead of being idle or pressuring your team members to engage with AI, I recommend taking a structured approach to get started.
- Education: It's important that every member of your organization understands how AI works. This includes different types of AI, models, and how they can be applied to day-to-day work. This will ensure all doubts among your staff members are removed, and they are comfortable accepting AI.
- Identify the key problem statements: This is absolutely critical. We need to understand the current set of problems that AI can help solve. Are we seeking cost optimization, or are we aiming to improve the customer experience? Do we want to reduce outages? Identifying and prioritizing your problem statements will allow you to narrow your scope and avoid getting overwhelmed.
- Validate: Once you have identified your problem statements, it's important to validate how AI can help. While AI is often seen as a genie today, it is not. It cannot solve all problems. Hence, validating which problems AI can solve and which it cannot is an important next step.
- ROI: Depending on which AI model you use to help resolve your problem, there will be a cost. For example, if you are going to use the OpenAI LLM model, it will cost you more compared to using Meta open-source models or other open-source models. In addition to the cost of using the models, you need to invest time in your people to learn and implement them. Hence, having a proper ROI analysis is important before you get started.
- Team: While there is no right answer here, I would recommend creating a small or pilot team to work on a couple of problem statements initially. Instead of involving everyone, a focused team will produce much faster results and can serve as an example for other teams.
I hope this article helps you get started on your AI journey.
Good luck!