By: Himanshu Chugh
Introduction
Every month, approximately 0.8 million leads visit a group of Rakuten Insurance websites. The marketing team aims to nurture high-potential leads to convert them into customers.
Lead nurturing is a crucial aspect of any successful marketing strategy. It involves building relationships with potential customers and guiding them through the sales funnel. Traditionally, businesses have relied on manual lead scoring methods that are often subjective and time-consuming to learn. With recent advances in machine learning, lot of research and practical applications in industry has been conducted to use ML models to predict the conversion propensity of leads. This conversion propensity score can be used to identify and prioritize the most promising leads for targeting through various marketing campaigns. Apart from just identifying the most promising leads, we can also use machine learning to segment All Leads into various stages of conversion funnel, such as Awareness, Interest, and Demand. Identifying such stages allows businesses to tailor their nurturing efforts (guide the design of ad creatives) and deliver highly personalized experiences to each lead to nurture them down the conversion funnel.
At Rakuten, we have taken a unique approach of Lead Nurturing. Along with predicting the propensity score of a lead to convert, we also match them with existing customers to understand the type of customer they would become. This dual information for each lead helps marketers design effective ad creatives. We have developed a family of ML models to build an end-to-end Lead Nurturing Solution for Rakuten Insurance
Lead Scoring Model
The lead scoring model uses machine learning to discover a business’s patterns of lead conversion. Based on these patterns, the model can predict conversion propensity of current leads. We treat this problem as a classification model where positive and negative labels are defined as below:
- Positive Label - Leads who browsed the website and converted during a pre-defined future horizon.
- Negative Label - Leads who browsed the website but did not convert during a pre-defined future horizon.
We engineered wide variety of features such as demographics, category interest and purchase on Rakuten Ichiba, points behaviour, and most importantly, page visit behaviour of leads on group of Rakuten Insurance websites.
Rakuten Insurance has wide variety of insurance products covering all essential needs like Medical, Car, Pet etc. We built a generalized model training pipeline that made it easier and faster for us to train and deploy lead scoring model for any insurance product, without going through the hassle of data preparation and feature engineering from scratch.
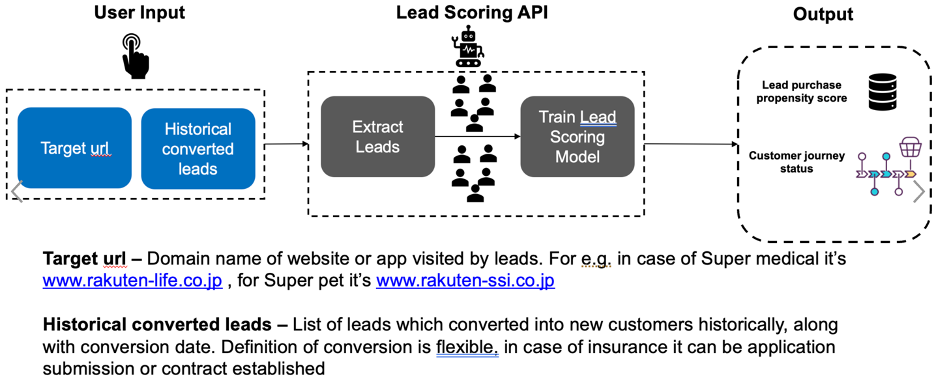
Leads are then segmented into conversion stages (Awareness, Interest, and Demand) based on their conversion propensity score.
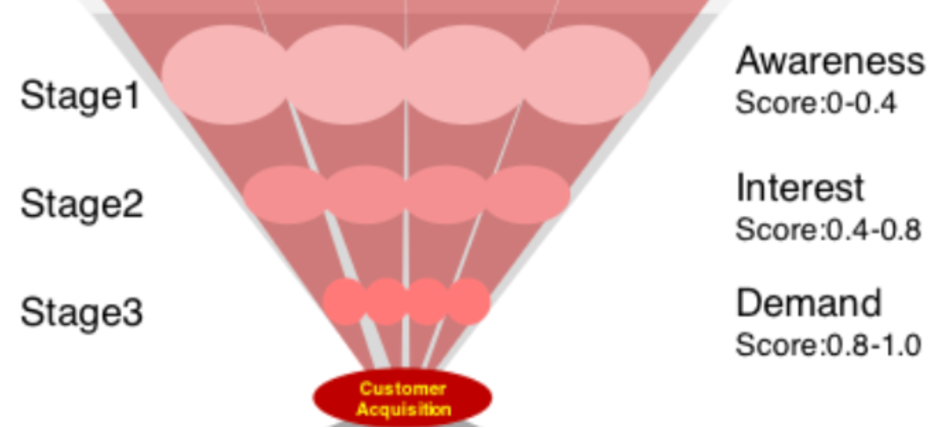
Similarity of Leads with Existing Customers
In this step our focus is to understand different segments within existing customers of the insurance product and then use each segment as seed list to find their lookalike within leads. This step can be broken down into two stages:
- Clustering of Existing Customers – A wide variety of information available for existing customers such as age, gender, location, policy type, claims history, add-ons purchased, medical history etc., can be used to segment them into various clusters. Unsupervised learning algorithms such as K-means clustering, hierarchical clustering, and DBSCAN can automatically identify patterns and similarities in customer data without the need for predefined labels or categories.
- Finding lookalike within Leads - Once the existing customers are segmented, each customer segment is used as a seed list and we use lookalike modelling to find leads which match with at least one of the seed list. There are multiple approaches to achieve this, we are highlighting two most common methods used in Industry:
- Classification Model-based - This approach uses seed list as positive labels and randomly selects negative labels from non-seed list user base. Wide variety of features (which are available for both seed and non-seed users) can be used for creating user representation or feature vector. Classifier models can be trained for each seed list. Every lead can be scored with each of the classifiers and assigned to a unique customer segment based on model score. One drawback of this approach is that classifier models perform well for seed lists that are sufficiently large, but they do not perform well for smaller seed lists.
- Similarity-based - Similarity-based methods outperform classifier-based baselines for small seed lists. This approach involves following two steps:
- Train Global User Embeddings - Techniques like word2vec, GloVe, or deep learning-based models can be used to generate embeddings for each user in the dataset. These models learn to represent users based on their attributes, behaviors, or preferences. Various discrete and continuous features can be used for generating user embeddings. Example of discrete features are user demographics, various pages visited on Insurance websites. Examples of continuous features include time spent on the website, days since last visit, etc.
- Similarity search - Once user embeddings are generated, we can use similarity metrics like "Cosine Similarity" and compare all pairs of seed users and available users among the leads, then determine look-alikeness based on distance measurements.
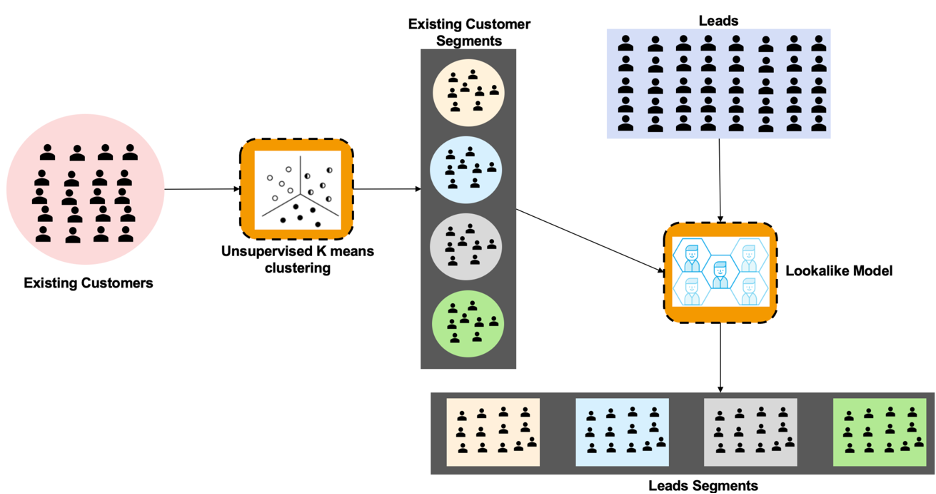
Putting it all together
As described above, for a given lead visiting our website, we have calculated the propensity to purchase score as well as the lookalike segment. This information can be used by new user acquisition teams to design effective and personalized ad creatives leading to an uplift in Conversion Rate as compared to targeting every lead with same content.
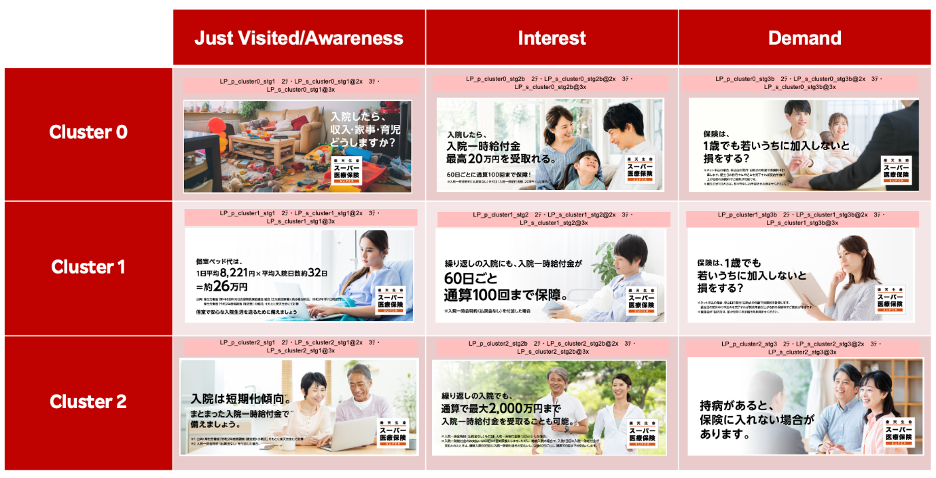
The Journey has Just Begun
There is much our team, our business stakeholders, and engineering partners have learned since we began building the Lead Nurturing Model. The entire pipeline, which consists of suite of ML models for Lead Scoring, Clustering, and Lookalike, has been deployed in production for Rakuten Insurance. Every week, millions of leads visiting our group websites are scored and shared with multiple marketing teams for targeting.
In this article we have only covered "Lead Nurturing" solution, which is part of a bigger umbrella of "Customer Nurturing", covering multiple Customer Targeting solutions for entire customer journey from the time they are a "Prospect" to becoming a "Loyal Customer."
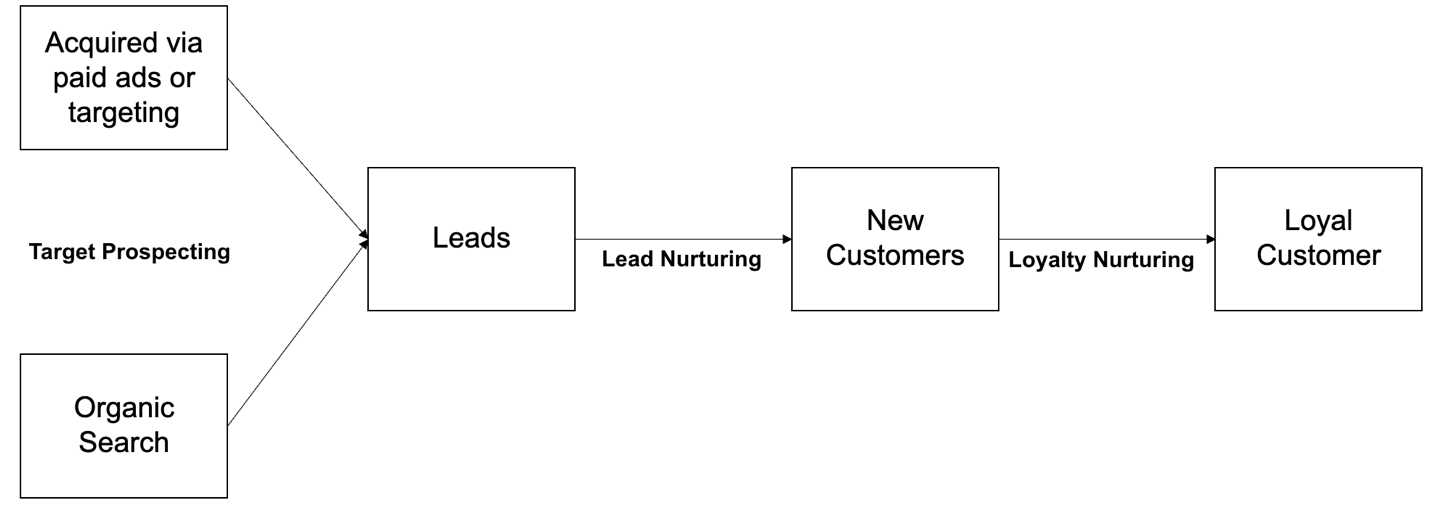
At Rakuten, we have developed and deployed range of ML solutions for Customer Targeting, which are leading to significant improvements in Customer Acquisition, Growth, and Retention Rates.
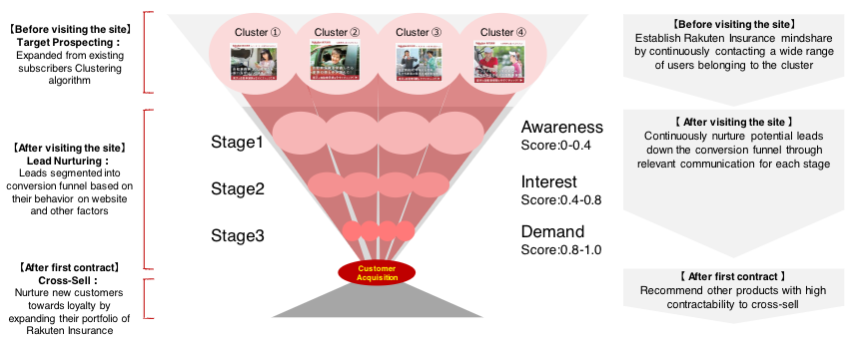
As a next step, we are planning to expand this solution to other Rakuten Business where a lead goes through a similar journey before becoming a customer.
Overall, it's always exciting to solve new challenges, and we'll keep experimenting with state-of-the-art methodologies to improve the performance of our ML models and deliver content that delights and inspires our customers, helping them find their favorite products effectively.