AI in BI: Empowering Smarter Data Narratives through Data Intelligence
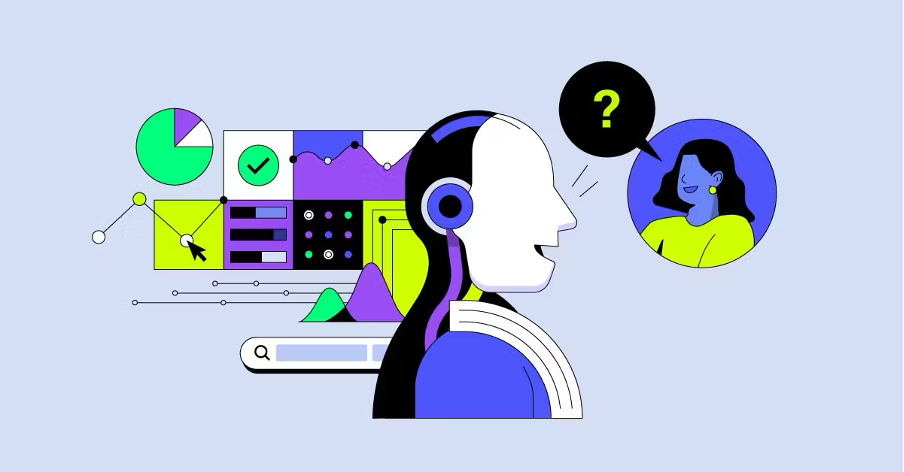
In today’s fast-paced, information-centric world, businesses are constantly seeking ways to extract deeper insights and make smarter decisions. Traditional Business Intelligence (BI) tools, while foundational, often fall short in meeting the evolving demands of modern organizations. But what if AI could bridge this gap? By integrating AI into BI, businesses can move beyond static dashboards and manual processes to unlock dynamic, actionable insights. In this blog, we explore how AI and Data Intelligence platforms are reshaping the BI landscape, empowering organizations to harness the full potential of their data and drive innovation.
The Current State of BI and the Need for AI: Challenges and Modernization
Business Intelligence (BI) has long been the backbone of data-driven decision-making, enabling organizations to analyze historical data and generate insights. However, traditional BI systems are increasingly falling short in meeting the demands of modern businesses. As organizations grow more complex and data volumes explode, these shortcomings have become glaringly apparent.
Traditional BI tools rely heavily on dashboards and static reports to deliver insights. While dashboards have been a staple of BI for decades, they often fail to answer evolving business questions. According to a Gartner report, 60% of BI users are dissatisfied with their dashboards, citing their inability to provide actionable insights or adapt to changing business needs.
Moreover, traditional BI systems depend heavily on data teams to create and maintain dashboards, reports, and queries. This reliance creates a bottleneck, as overworked data teams struggle to keep up with the growing demand for insights. A McKinsey report found that data analysts spend up to 80% of their time on manual data preparation tasks, leaving little time for strategic analysis. This inefficiency not only delays decision-making but also limits the ability of business users to explore data independently.
Evolution of Data Platforms: The Story so Far
The journey of data platforms has evolved significantly over the decades to meet the growing complexity of enterprise data needs. From data warehouses to data lakes, and more recently to data lakehouses, the evolution of data platforms has combined the best of both worlds offering a unified system to handle structured and unstructured data for both BI and AI workloads.
Despite these advancements, current platforms face significant challenges. Technical skill barriers (e.g., SQL, Python) limit accessibility for non-technical users, while data accuracy and curation issues make it difficult to find reliable data. Management complexity and rising costs strain resources, especially as data volumes grow. Additionally, governance and privacy concerns have become more pressing with evolving regulations in India, such as the Digital Personal Data Protection Act (DPDP) 2023.
From Challenges to Possible Solutions: The Role of Data Intelligence Platforms
Real-world Use Case: Addressing Metric Inconsistencies
For example, the definition of "service usage lift", a metric to evaluate the change in spending behaviour in a service due to the usage of another, might vary across different business units. A user viewing a dashboard that aggregates this data would likely assume they are comparing apples to apples, when that isn’t the case. This inconsistency can lead to misinterpretation of insights and flawed decision-making.
Now imagine if a BI tool were intelligent enough to detect these discrepancies automatically. Instead of presenting the user with misleading aggregated data, the tool could flag the differences in definitions and provide a solution. For example, offer a contextual explanation to the user, highlighting how the calculation differs and what adjustments might be needed for a fair comparison. This level of intelligence would ensure that users are equipped with accurate, actionable insights and prevents costly errors stemming from inconsistent data interpretations.This example highlights the potential of AI to address inconsistencies in BI.
The Promise of AI in BI
The advent of Artificial Intelligence (AI) has sparked hope for a more efficient and accessible BI landscape. For example, tools like Databricks Assistant allow users to generate SQL queries and create dashboards using conversational language, empowering non-technical users to interact with data directly.AI can also automate repetitive tasks, such as data preparation and query generation, freeing up data teams to focus on high-value initiatives. A case study by ThoughtSpot demonstrates this potential: Wellthy, a healthcare company, used ThoughtSpot’s natural language search to empower its care team to analyze real-time patient data without relying on data teams. This shift saved the company over $200,000 by increasing analyst efficiency.
Rakuten has been at the forefront of leveraging AI to empower its employees, or "Rakutenians," through its AI-nization efforts. These initiatives aim to integrate AI into the daily workflows of all employees, enabling them to better understand and utilize AI tools for decision-making and problem-solving. For instance, Rakuten has developed internal AI tools like ‘Rakuten AI for Rakutenians’ that allow employees to leverage AI in all facets of their daily tasks, democratising access to AI.
Keyways organizations are using AI to augment BI, analytics, and data management:
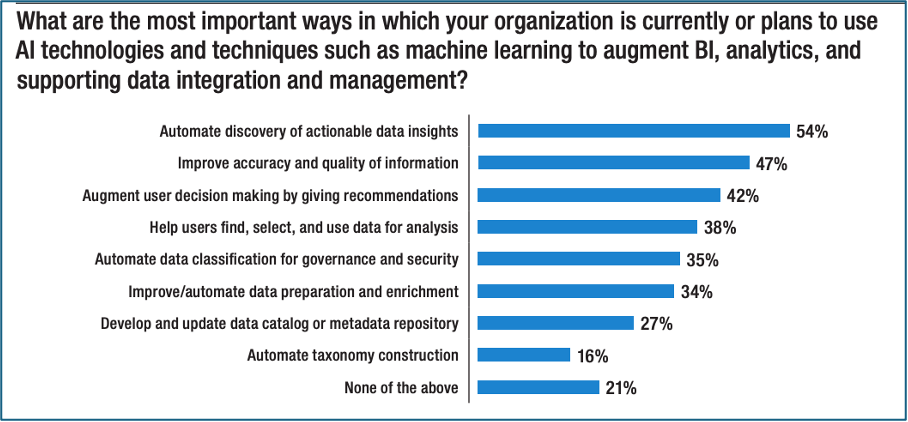
Source: Hitachi Vantara, "BI and Analytics in the Age of AI and Big Data."
Despite its promise, generative AI (GenAI) has struggled to deliver meaningful results in BI. Many current AI implementations rely on solutions that attempt to layer AI capabilities onto existing BI tools. However, these solutions often fail when faced with messy data, ambiguous language, and the nuanced complexities of real-world data analysis. For example, simply pointing a large language model (LLM) at a database schema and using text-to-SQL often produces inaccurate or incomplete results because the schema lacks critical business context. As a result, many organizations find that GenAI solutions fall short of their expectations, leaving critical questions unanswered.
Key Features and Customer Use Cases of DI Platforms
As organizations grapple with the growing complexity of their data ecosystems, Data Intelligence (DI) platforms have emerged as a transformative solution. Unlike traditional BI tools, which focus on visualizing data, DI platforms leverage AI models to deeply understand the semantics of enterprise data—its meaning, context, and usage. At its core, Data Intelligence is about making data smarter. DI platforms use AI to analyze not just the data itself but also its metadata, lineage, and usage patterns.
-
Natural Language Access: DI platforms enable users to interact with data using natural language queries tailored to their organization’s jargon. For instance, a sales manager can ask, "What are the top-performing services this quarter?" and receive instant insights without needing technical expertise.
-
Semantic Cataloging and Discovery: DI platforms create a semantic catalog of enterprise data, making it easier to search, discover, and explore datasets. They also identify discrepancies in data usage, ensuring consistency across the organization.
-
Automated Management and Optimization: By analyzing data workflows, DI platforms optimize data storage, indexing, and partitioning, reducing costs and improving performance.
-
Enhanced Governance and Privacy: DI platforms enforce governance policies, track data lineage, and protect sensitive information, ensuring compliance with regulations.
-
Seamless Integration with AI Workloads: DI platforms bridge the gap between AI and BI by providing AI models with the context and semantics they need to deliver accurate results.
Unlike traditional BI tools, DI platforms provide a holistic view of data usage and lineage, enabling organizations to manage and optimize their entire data lifecycle. This makes DI platforms not just a tool for analysis but a comprehensive solution for data management, governance, and AI integration.
The Future of AI in BI: Trends, Challenges and Closing thoughts
The future of Business Intelligence (BI) is being shaped by the rapid advancements in Artificial Intelligence (AI), which are transforming how organizations interact with and leverage their data. As businesses strive to become more data-driven, AI is poised to play a central role in making BI smarter, faster, and more accessible with several key trends shaping the future:
Emerging trends highlight the growing role of AI in BI. One such trend is the Rise of Conversational BI Tools Powered by LLMs, where Large Language Models (LLMs) enable conversational BI tools that allow users to interact with data using natural language, empowering non-technical users to explore data independently. Increased Adoption of Data Intelligence (DI) Platforms, which are becoming the backbone of modern BI systems is another. These platforms offer a unified solution for managing, analyzing, and optimizing data, seamlessly integrating AI into BI workflows, driving widespread adoption across industries, and enabling organizations to unlock the full potential of their data.
However, challenges remain. A critical challenge is Ensuring Data Accuracy and Reliability, as AI-driven systems are only as good as the data they analyze. This makes data quality, accuracy, and curation critical priorities for organizations. Additionally, Balancing Innovation with Ethical Considerations is essential, as AI becomes more integrated into BI. Ethical concerns around data privacy, security, and bias must be addressed, and implementing robust governance frameworks is key to ensuring responsible AI usage while balancing innovation with ethical considerations.
Closing Thought
The future of BI lies in harnessing the power of AI to transform data into actionable insights. By addressing the challenges and embracing innovation, organizations can unlock the full potential of their data and drive meaningful change. Data Intelligence platforms, with their ability to deeply understand the semantics and context of enterprise data, will play a pivotal role in bridging the gap between raw data and actionable insights.As AI continues to evolve, it will not only enhance BI but also redefine how businesses operate, making data-driven decision-making a cornerstone of success.
References