Breaking Down Data Silos: How AI-Augmented Data Engineering Powers a 360° Customer View in E-Commerce
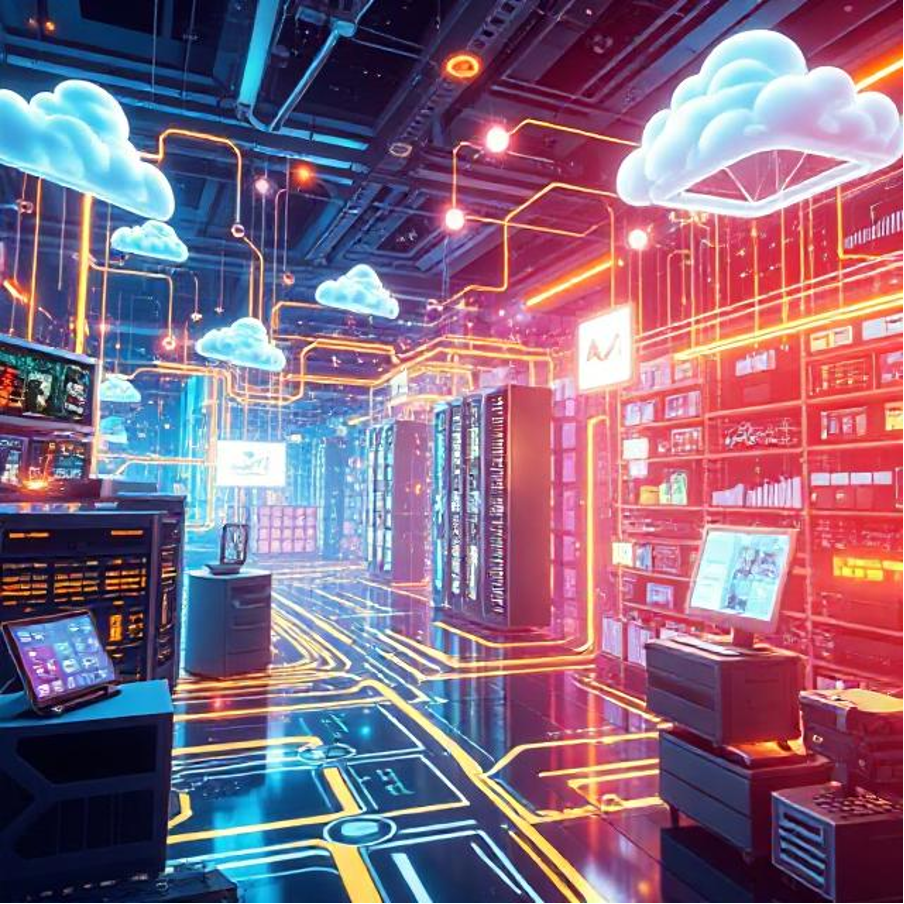
Imagine this: You're running an e-commerce business, and your marketing team is sending out ads and campaigns every day. But instead of delighting your customers, you're frustrating them. Some users get promotions for products they've already purchased, others see offers for things they don't care about, and a few unlucky ones are so bombarded with irrelevant emails that they unsubscribe altogether. Sound familiar? That was us—drowning in data but struggling to make sense of it.
We knew we had a goldmine of information—browsing history, sales data, user demographics, and more—but we weren't using it effectively. Our campaigns, while technically “personalized,” felt generic. Engagement was low, costs were spiraling, and worst of all, we were missing opportunities to truly connect with our customers.
It was clear we needed a change. So, we made the leap to the cloud. But not just any cloud migration—we embraced AI-augmented data engineering to optimize our systems. This transition helped us create a unified, 360-degree view of our customers, automate manual tasks, ensure data quality, and use AI to make quicker, smarter decisions.
Here's the story of how we turned chaos into clarity, reduced campaigns by 30%, cut costs, and increased sales—all by leveraging the power of AI in the cloud to level up our data engineering, infrastructure, and decision-making.
The Problem: Too Much Data, Too Little Insight
In e-commerce, data is everywhere. Every click, search, and abandoned cart tells a story. But when you're dealing with millions of users and products, those stories can quickly turn into noise. That's exactly what we were facing:
- Browsing History: Users explored products across categories, but we couldn't pinpoint their true interests.
- Sales Data: We had mountains of transaction data but lacked the ability to predict what users might buy next.
- Campaigns: We rolled out campaigns based on basic data, hoping for higher conversion rates. Spoiler alert: It didn't work.
- Customer Loyalty Data: We tracked frequent buyers through points and rewards but failed to leverage this data effectively.
The result? Low engagement, high costs, and frustrated users. We needed a way to cut through the noise and deliver smarter, more effective campaigns. That's when we turned to AI-augmented data engineering.
The Turning Point: Building a Smarter Data System
The first thing we realized? Our data was a mess. It was scattered across multiple systems, full of inconsistencies, and riddled with gaps. Before we could even think about using AI, we had to get our data in order.
We adopted a Data Lakehouse strategy, which combines the flexibility of a data lake with the structure of a data warehouse. Our teams split into two groups to tackle this:
- Data Lake Team: Focused on building an AI-powered data ingestion framework with high levels of automation and reliability.
- Data Warehouse Team: Focused on automating ETL processes, implementing robust data monitoring systems, and creating self- healing pipelines to keep everything running smoothly.
The result? A 40% improvement in efficiency across the board. With our data finally organized, we were ready to supercharge our operations with AI.
The Solution: How AI Helped Us Unlock Real-Time Insights
Here's how we used AI to solve our problems and transform our business:
-
AI-Powered Cloud Infrastructure Management
Moving to the cloud was step one. It gave us the scalability we needed, and AI made it even better.
- Predictive Scaling: AI predicted traffic spikes (like during sales or promotions) and automatically adjusted cloud resources to handle the load.
- Anomaly Detection: AI monitored our infrastructure in real time, catching performance issues before they became problems.
- Optimized Resource Usage: AI analyzed cloud usage and optimized resource allocation, keeping costs low and performance high.
The result? We could scale seamlessly, improve performance, and keep costs under control.
-
Seamless Code Migration from On-Prem to Cloud
Migrating our legacy code to the cloud could've been a nightmare. But AI made it a breeze.
- Smart Query Builder: AI scanned our old code, identified what needed to change, and automatically refactored it for the cloud.
- Faster Deployment: AI automated the deployment process, resolving issues quickly and ensuring a smooth migration.
The result? We moved to the cloud faster, with fewer errors, and ended up with more efficient queries.
-
Proactive Error Detection and Self-Healing Pipelines
Good data is the foundation of great customer insights. AI helped us keep our data clean, accurate, and reliable.
- Data Quality Monitoring: AI continuously monitored our pipelines, flagging issues like delays or schema mismatches in real time.
- Self-Healing Pipelines: AI tools automatically fixed common issues, like retrying failed jobs or correcting schema mismatches.
The result? We maintained high-quality, trustworthy data, which was crucial for building a reliable 360-degree customer profile.
The Impact: Turning Data into Actionable Insights
With our data unified and AI in place, we started seeing real results:
-
Data-Driven Product Decisions
Unified data helped us spot trends and make better product decisions.
Example: By analyzing browsing and sales data, we noticed a growing interest in eco-friendly products. This led to the launch of a new product line, which became a top seller.
Impact: Revenue from the new product line exceeded projections by 40%. -
Holistic Customer Segmentation
With richer data, we created more meaningful customer segments.
Example: Instead of basic demographic segments, we built behavior- based ones like “frequent buyers,” “bargain hunters,” and “window shoppers.”
Impact: Engagement improved across the board, and cross- functional teams were better aligned.
The Takeaway: From Siloed Data to Unified Insights
Our journey from fragmented data to a unified platform wasn't just about technology—it was about transforming how we worked as a team. By leveraging AI-augmented data engineering and cloud tools, we turned customer data into a shared asset that drives collaboration and delivers measurable results.
If your organization is struggling with siloed data, take it from us: The key isn't just collecting data—it's unifying and using it effectively. With the right foundation, the possibilities are endless.
What's Next: AI-Powered Chatbot for Real-Time Data Access
We're not stopping here. One of our most exciting projects is an AI- powered chatbot that gives business teams instant access to data—no coding required.
- Natural Language Processing (NLP): The chatbot understands plain-language questions like “What are today's top products?” or “How are sales in the women's category this week?”
- Instant Insights: Connected to our cloud-based data warehouse, the chatbot pulls real-time data on customer profiles, sales, and inventory.
- Self-Service Analytics: Business users can get insights on demand, without waiting for developers.