CAG vs RAG: Which AI Approach Reigns Supreme in 2025?
In the rapidly advancing field of artificial intelligence, Large Language Models (LLMs) are pivotal, especially for applications requiring document retrieval to enhance their capabilities. Retrieval-Augmented Generation (RAG) has emerged as a popular technique, combining the power of LLMs with the ability to access external data in real-time, addressing the limitation of classic LLMs that can only work with information available at the time of their training. RAG enables access to up-to-date and accurate information by retrieving external knowledge during inference, grounding outputs in real-world data. However, RAG is not without its limitations. It can introduce latency due to real-time searches and requires maintaining a complex retrieval pipeline, potentially leading to errors in document selection. Cache-Augmented Generation (CAG) has emerged as a significant competitor to RAG. CAG enhances efficiency and context retention by storing and reusing relevant outputs. It preloads all the information into the model's memory, eliminating the search step and providing direct access to cached knowledge for instant answers.
Here's a comparison of the two approaches :
Feature |
Retrieval-Augmented Generation (RAG) |
Cache-Augmented Generation (CAG) |
---|---|---|
Data Handling |
Dynamically retrieves documents |
Preloads all relevant documents |
Speed |
Slower due to real-time retrieval |
Up to 40x faster inference |
Complexity |
More complex due to retrieval pipeline |
Simplified architecture |
Knowledge Source |
External database or search engine |
Preloaded into model context |
Best Use Cases |
Dynamic or large datasets |
Static knowledge bases |
Error Vulnerability |
Prone to retrieval and ranking errors |
No retrieval errors |
Response Time |
Slower due to retrieval latency |
Faster due to preloaded knowledge |
Scope of Knowledge |
Dynamic and large-scale |
Static and limited to preloaded data |
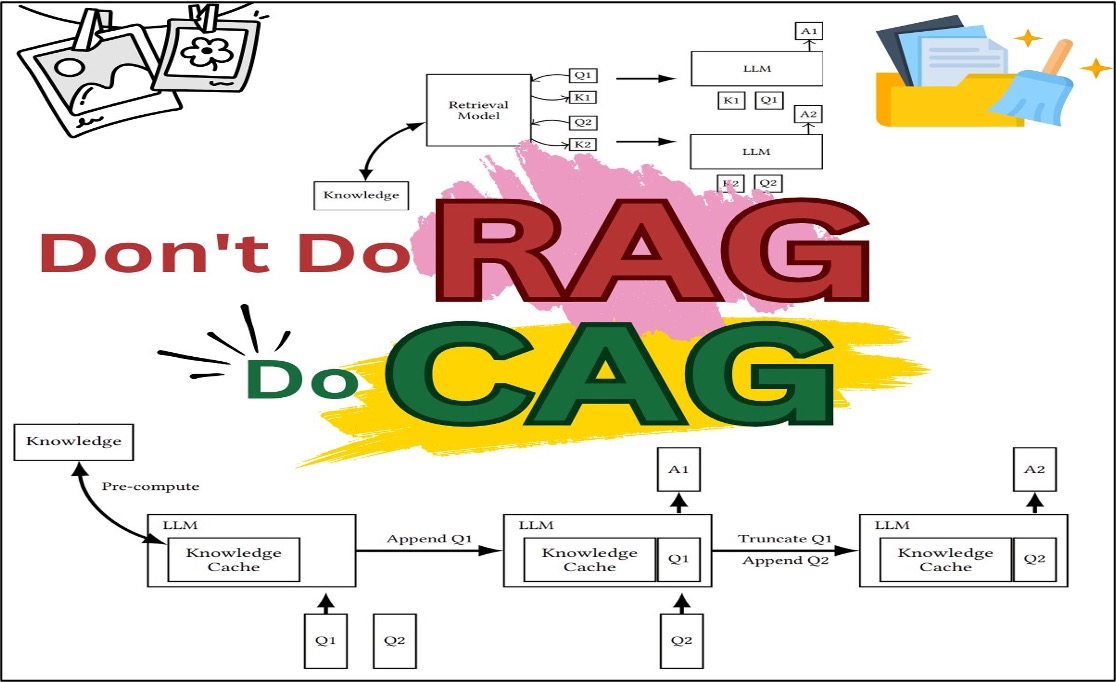
Advantages and Disadvantages :
RAG |
CAG |
|
---|---|---|
Advantages |
Scalability: Handles vast datasets without preloading all information. Flexibility: Fetches only relevant data at runtime. Broad Application Range: Effective for unpredictable queries. |
Speed: Preloaded context allows for instant responses. Accuracy: Holistic processing of data ensures more precise answers. Simplicity: Fewer components lead to easier maintenance. |
Disadvantages |
Latency Issues: Real-time searches can slow down response times. Complex System Maintenance: Requires ongoing management of retrieval processes. Document Selection Errors: Risks providing incorrect or irrelevant information. |
Context Limitations: Limited by the model's context window, which can restrict data size. Costly for Large Datasets: Requires significant memory for caching. Potential Irrelevance: Risk of including unnecessary information in responses. |
When to Use Each Approach :
RAG |
CAG |
|
---|---|---|
When |
You need access to a large or constantly updated dataset. Flexibility in retrieving relevant information is critical. Your application involves open-ended queries where scope is unpredictable. |
Your knowledge base fits within the model's context window. You require extremely fast responses. Information is stable and does not change frequently. |
RAG Architecture :
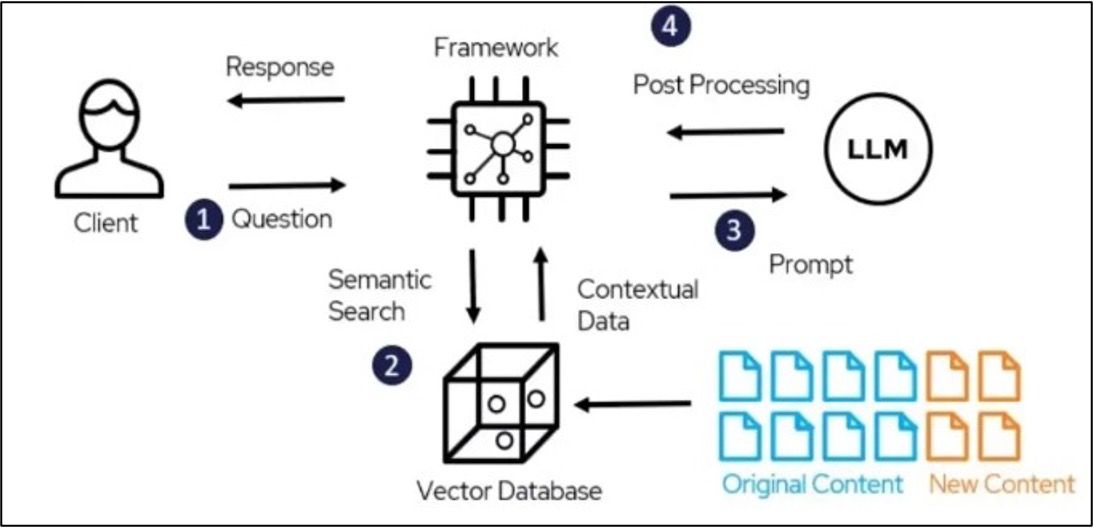
RAG architecture bridges vector search and LLMs to deliver contextually rich, retrieval-augmented answers.
CAG Architecture :
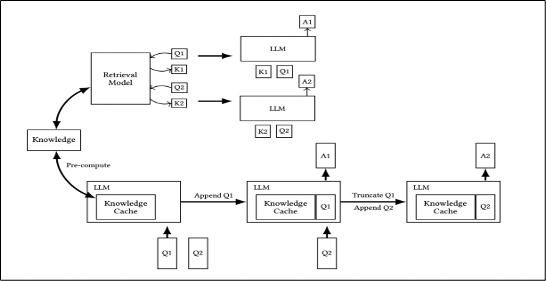
CAG architecture integrates a retrieval model and an LLM with a knowledge cache to iteratively refine and provide context-aware responses.
The choice between CAG and RAG depends on the specific needs of the application. CAG is highly recommended when the main requirement is to maximize speed and reduce dependencies on external systems, especially in static domains where all relevant information can be preloaded without major issues. RAG becomes the most suitable alternative if the priority is to have real-time information with constantly updated data.
Future Scope
The potential for combining CAG with RAG in a hybrid approach exists, where frequently accessed information is in CAG for instant access, while RAG handles more rarely needed details. As LLMs improve at managing vast contexts with lower costs, CAG could become the default for most projects due to its efficiency. However, RAG will likely remain indispensable for edge cases with exceptionally large or highly dynamic knowledge bases.
References