Customisation and Optimisation of Large Language Model
Introduction
Large Language Models (LLMs) represent a transformative leap in artificial intelligence. Models like OpenAI’s GPT, Meta’s Llama, and Mistral are designed to generate and understand human-like text with remarkable versatility. Their popularity stems from their ability to learn and adapt across multiple tasks without requiring retraining for each new application. Traditional AI/ML models often need extensive domain-specific training and are restricted in scope, whereas LLMs, trained on vast datasets, are inherently more powerful and general-purpose.
A significant strength of LLMs is their capacity to process context effectively. They generate meaningful responses by understanding the broader nuances of a query, enabling applications like intuitive chatbots and automated document drafting. Traditional models typically lack this level of contextual awareness, giving LLMs a clear advantage. The range of tasks LLMs can perform continues to expand. They excel in summarizing information, analyzing sentiment, extracting data, writing code, answering questions, translating languages, drafting essays, coding, engaging in conversations and even synthesizing knowledge for scientific research. Their adaptability makes them valuable across sectors such as healthcare, finance, education, and entertainment, where they solve problems and enhance productivity.
As industries continue to explore their potential, LLMs are poised to become a cornerstone of modern technology, influencing how we interact with AI in both professional and everyday contexts.
Closed Source LLMs Vs Open Source LLMs
Historically, closed-source models developed by organizations like OpenAI and Google have led in performance due to substantial investments in research and infrastructure. However, open-source models are rapidly advancing. For instance, Meta's Llama series has demonstrated significant improvements, with Llama 3.1 showing capabilities comparable to leading closed-source models. The rise of open-source LLMs offers several advantages:
-
Transparency: Open-source models allow researchers and developers to inspect and understand the underlying architecture and training data, fostering trust and facilitating improvements.
-
Customization: Organizations can fine-tune open-source models to cater to specific domains or applications, enhancing relevance and performance for particular use cases.
-
Cost Efficiency: Utilizing open-source models can reduce costs associated with licensing fees of proprietary models, making advanced AI capabilities more accessible to a broader audience.
Reference:
As open-source LLMs continue to evolve, they are closing the performance gap with closed-source counterparts, promoting innovation and collaboration within the AI community.
Why Customisation is Needed in LLMs?
While open-source LLMs like Llama and Mistral are indeed fine-tuned before release, they are optimized for general-purpose tasks across a wide range of topics. Customization and optimization are necessary to address specific business needs, domain requirements, or operational constraints. Here's why:
Domain-Specific Expertise
-
Pre-trained LLMs are generalists and may not perform well on niche topics or specialized industries.
-
Customizing allows the model to become proficient in a specific domain, like law, medicine, or finance.
-
Examples
-
Hugging Face’s BLOOMZ demonstrates the power of domain-specific customization by fine-tuning the general-purpose BLOOM model for multilingual and cross-lingual tasks. Leveraging curated datasets, BLOOMZ excels in low-resource languages, ensuring accurate translations, context retention, and cultural sensitivity. This specialization benefits industries like healthcare, law, and e-commerce, enabling tasks such as translating health guides into rare languages, summarizing multilingual legal contracts, and generating localized product descriptions. By optimizing for translation accuracy and cross-lingual understanding, BLOOMZ enhances accessibility, global collaboration, and user engagement, making it invaluable for multilingual and culturally diverse applications.
-
Domain-specific customization of Large Language Models (LLMs) like MedPaLM in healthcare has revolutionized the field by addressing critical needs with precision and efficiency. MedPaLM, fine-tuned with medical datasets such as PubMed and MedQA, assists in clinical decision support by suggesting diagnoses and treatments, enhances telemedicine consultations by providing evidence-based recommendations, and simplifies medical literature for doctors and researchers. It automates clinical documentation, generates patient-friendly explanations of medical information, and ensures safety through drug interaction alerts. These applications reduce diagnostic errors, streamline administrative tasks, empower patients, and improve access to quality healthcare, demonstrating the transformative impact of domain-specific LLM
-
Domain-specific customization of Large Language Models (LLMs) in the financial domain has significantly enhanced efficiency, accuracy, and decision-making. BloombergGPT, a closed-source LLM fine-tuned on extensive financial datasets, excels in addressing specific challenges in the industry. It assists in fraud detection by identifying suspicious transactions, enhances risk analysis through tailored market and credit assessments, and supports automated compliance reporting to ensure adherence to regulatory standards like KYC and AML. Additionally, it streamlines investment research by analyzing financial reports, market data, and trends, and provides personalized financial insights for improved portfolio management. By automating routine tasks and delivering actionable insights, BloombergGPT drives operational excellence and transforms financial decision-making.
-
Task-Specific Performance
-
Open-source LLMs are not optimized for specific tasks like sentiment analysis, legal reasoning, or summarization.
-
Fine-tuning aligns the model with the particular format, vocabulary, and nuances of the target task.
-
Examples:
-
Mistral 7B LLM has difficulty reliably generating well-structured JSON outputs. By fine-tuning it on a curated dataset of labelled JSON examples, one can enhance its ability to produce consistent, accurate, and properly formatted JSON responses tailored to specific needs
-
Task-based customization of LLMs enhances their ability to excel in specialized applications by aligning them with task-specific formats, vocabulary, and nuances. For instance, Claude by Anthropic, fine-tuned for customer support tasks, enables efficient resolution of complex queries such as refund policies, order tracking, and troubleshooting. This customization reduces response times, improves customer satisfaction, and minimizes the need for human intervention in repetitive queries.
-
Similarly, Codex by OpenAI, fine-tuned for structured code generation, is optimized to produce accurate and well-structured outputs in JSON or Python. This targeted customization enhances developer productivity, accelerates prototyping, and minimizes coding errors, making it an invaluable tool for software development teams. These examples demonstrate how task-based fine-tuning unlocks the full potential of LLMs across diverse industries.
-
Operational Constraints
-
Large models are computationally expensive, requiring high-end hardware for inference.
-
Customization can involve model distillation or low-rank adaptation (LoRA) to reduce size and improve efficiency, enabling deployment on edge devices or resource-limited environments.
-
Examples:
-
Meta’s Llama 2-Chat: Although Llama 2 was general-purpose, its fine-tuned variant (Llama 2-Chat) was specifically adapted for conversational AI, making it more efficient for chat-based applications.
-
Operational constraints often make large LLMs unsuitable for deployment on resource-limited environments. Fine-tuned models like LLaMA 2-Chat, optimized using low-rank adaptation (LoRA), address this by reducing size and improving efficiency. For example, LLaMA 2-Chat is deployed on edge devices in smart home systems to power voice assistants for tasks like controlling thermostats and lighting. This reduces latency, enhances user privacy through local processing, and minimizes reliance on cloud resources.
-
Similarly, models like Mistral 7B, distilled into smaller, efficient variants, enable on-device personalization for smartphones. These models handle tasks such as email composition, app recommendations, and content summarization directly on the device. This ensures functionality even in areas with limited internet connectivity, lowers energy consumption, and enhances user experience by providing faster and more reliable AI capabilities.
-
Benefits of Customisation
-
Increased Accuracy: Tailoring the model improves relevance and precision in specialized use cases.
-
Better User Experience: Models customized for specific languages, dialects, or cultural contexts provide more natural and localized interactions.
-
Cost Efficiency: Optimized models require fewer computational resources for inference, reducing operational costs.
Customising the Open Source LLMs
Pre-requisites
Data Collection for the Target Subject
-
Finding Existing Datasets: Explore platforms like HuggingFace for publicly available datasets relevant to your subject (E.g: open-web-math, starcoderdata). Data might not be available free of cost and one might need to purchase it.
-
Data Scraping: Use crawler-based solutions to gather data from missing or less accessible sources (E.g: fineweb).
-
Leveraging Higher-Order LLMs: Utilize advanced language models to generate or curate datasets for training a smaller, task-specific model.
-
Reference: https://arxiv.org/abs/2306.15895
-
-
Data Transformation: Convert the data file into a format compatible with the model, such as JSON or JSONL.
-
Model Deployment: Deploy the model on NVIDIA GPUs for optimal performance and efficiency. Details of the actual deployment steps on Kubernetes cluster can be found in the articles shared below:
Reference:
Steps for Customizing Open-Source Models
Customization of Open-Source models involves two steps namely pre-training followed by fine-tuning.
Think of pre-training and fine-tuning like learning a language:
-
Pre-Training: Imagine learning English by reading novels, newspapers, and websites. You understand grammar and vocabulary broadly but aren’t an expert in any particular topic.
-
Fine-Tuning: Now, you take a course on medical English. You learn terms like "myocardial infarction" or "cardiovascular system" to become proficient in conversations related to healthcare.
This two-step process ensures the model is both versatile and capable of handling specific tasks effectively.
-
Pre-Training
This is the first step where the model learns a general understanding of language by processing vast amounts of data. Here you can choose any language.
-
Objective:
-
Teach the model the basic structure, syntax, and patterns of language.
-
Enable it to predict the next word in a sentence or fill in missing text.
-
-
What Happens:
-
The model is trained on large-scale datasets (e.g., books, articles, websites) to recognize language patterns.
-
It learns general language rules without focusing on any specific domain.
-
For example:
-
Given "The cat sat on the ______", the model learns that "mat" is a likely next word.
-
-
The model adjusts billions of parameters (mathematical values) to improve its predictions over time.
-
-
Example:
-
If you train an open-source model like Mistral 7B on Wikipedia, it will learn a wide array of topics but not specialize in any.
-
-
Time taken:
-
Llama 3.1 models required extensive computational resources, with training time dependent on model size and hardware infrastructure.
-
GPU Hours: Training a 7B parameter model on 1 TB of data over 3 epochs with 100 A100 GPUs is estimated to take 7–9 days, assuming an efficient pipeline and software stack (e.g., using libraries like DeepSpeed or PyTorch Fully Sharded Data Parallel).
-
Training Duration depends on following factors:
-
Model Size:
-
Number of parameters (e.g., 7B, 13B, 175B).
-
Larger models require significantly more computation per training step.
-
-
Dataset Size:
-
Number of tokens or total size of the training data (e.g., 1 TB).
-
More data increases the number of training steps.
-
-
Number of Epochs:
-
Total passes over the training data.
-
Increasing epochs extends training time.
-
-
Batch Size:
-
Number of tokens processed per forward and backward pass.
-
Larger batches require more memory and computational resources but reduce the number of steps.
-
-
Sequence Length:
-
Length of input text sequences.
-
Longer sequences increase computation per token.
-
-
GPU Type and Performance:
-
GPU hardware (e.g., A100, H100).
-
Performance metrics like TFLOPs and memory bandwidth.
-
-
Number of GPUs:
-
Size of the GPU cluster.
-
More GPUs enable parallelism, reducing time.
-
-
Precision Used:
-
Training precision (e.g., FP32, FP16, BF16).
-
Lower precision improves speed without significant accuracy loss.
-
-
Training Frameworks and Optimizations:
-
Use of frameworks like PyTorch, TensorFlow, DeepSpeed, or Hugging Face Accelerate.
-
Optimizations like mixed-precision training, model parallelism, and gradient accumulation.
-
-
Communication Overheads:
-
Latency and bandwidth between GPUs in multi-node setups.
-
Efficient communication protocols (e.g., NCCL) reduce bottlenecks.
-
-
Learning Rate and Scheduling:
-
Choice of optimizer and learning rate schedule.
-
Poorly tuned parameters can increase convergence time.
-
-
Data Loading and Preprocessing:
-
Efficiency of the data pipeline.
-
Slow I/O or preprocessing can bottleneck GPU utilization.
-
-
Checkpointing and Fault Tolerance:
-
Frequency of saving model checkpoints.
-
Frequent checkpoints add overhead but improve recovery in case of failure.
-
-
Software Stack and Libraries:
-
Compatibility and efficiency of libraries used (e.g., CUDA, cuDNN).
-
Better libraries leverage hardware capabilities effectively.
-
-
Training Objectives:
-
Complexity of the task (e.g., causal language modeling, masked language modeling).
-
Some objectives require more computation.
-
-
-
Reference:
-
-
Fine-Tuning
This is the customization step where the model is adapted to a specific domain or task for a targeted language.
-
Objective:
-
Teach the model how to perform well on a specific task or domain (e.g., medical terminology, legal documents).
-
-
What Happens:
-
A smaller, specialized dataset relevant to the target task is used (e.g., Japanese legal documents).
-
The pre-trained model is further trained using this dataset, with a focus on accuracy and relevance for the specific domain.
-
The model's parameters are fine-tuned to improve performance on domain-specific questions or applications.
-
Example task:
-
Input: "What is the penalty for breaching a Japanese contract?"
-
Expected Output: "Under Article X, the penalty includes fines up to Y yen."
-
-
Example task:
-
Input: "What are the tax implications of selling residential property in India?"
-
Expected Output: "The sale of residential property in India may attract capital gains tax. If held for more than 24 months, it qualifies as long-term capital gain, taxed at 20% with indexation benefits."
-
-
-
Example:
-
Fine-tuning the same Mistral 7B model with Japanese legal documents helps it become an expert in answering legal queries specific to Japan.
-
Fine-tuning the model for structured JSON output responses
-
-
Time taken:
-
Fine tuning is computationally very less expensive and takes much lesser time than training as the finetuning specific data is way smaller than to training data and fine tuning does not involve updating all the parameters of the model. It freezes the parameters and creates one layer of weights.
-
-
Testing & Benchmarking
Testing
The LM Harness Benchmarkis a tool designed to evaluate the performance of language models (LMs) across various tasks. It provides a standardized way to measure a model’s capabilities on different datasets and benchmarks, enabling comparisons between models on a wide range of linguistic and reasoning challenges. The reference link explains the required steps to carry out evaluation across different benchmarks.
Key Features of LM Harness:
-
Task Variety:
Covers tasks such as natural language inference, question answering, common-sense reasoning, factual knowledge assessment, and more.
Includes benchmarks like MMLU, HellaSwag, ARC, TruthfulQA and others
-
Customizable Evaluation:
Allows users to evaluate LMs on specific datasets or customize tasks for their particular needs.
Provides options for evaluating open-source and proprietary models.
-
Standardized Metrics:
Reports metrics like accuracy, perplexity, and F1 scores to assess model performance across different domains.
-
Ease of Use:
Simple interface for running evaluations on various models without requiring extensive coding or customization.
-
Comparison Across Models:
Enables users to benchmark multiple LMs, facilitating objective comparisons between open-source models like Mistral, Llama, or Falcon and proprietary models like GPT or Claude.
LLM Benchmarks
Evaluating the performance of Large Language Models (LLMs) as well as customised LLMs involves several key metrics and benchmarks that assess various aspects of their capabilities. These evaluations consider factors such as model size, response time, accuracy, and language support. Notable benchmarks include:
-
MMLU (Massive Multitask Language Understanding): Assesses a model's proficiency across a wide range of subjects, testing its general knowledge and reasoning abilities.
-
MMLU Pro: Massive Multitask Language Understanding - Professional (MMLU-Pro) features a comprehensive set of expertly curated multiple-choice questions designed to assess knowledge across diverse professional domains. These include areas such as medicine and healthcare, law and ethics, engineering, and mathematics, ensuring robust coverage and depth for evaluating domain-specific expertise.
-
GPQA: Graduate-Level Google-Proof Q&A (GPQA) is centered on PhD-level multiple-choice questions designed to test advanced knowledge in science. It encompasses a range of disciplines, including chemistry, biology, and physics, ensuring a thorough evaluation of expertise at the graduate level.
-
HumanEval: The HumanEval benchmark is designed to assess the code generation capabilities of large language models (LLMs). It consists of a curated set of programming problems that require models to generate functional Python code based on problem descriptions. The benchmark evaluates the correctness of generated solutions through unit tests, offering a reliable measure of an LLM's ability to understand instructions and produce accurate, executable code.
-
GSM-8K: The GSM-8K benchmark evaluates large language models (LLMs) on their ability to solve grade-school-level mathematical word problems. It consists of 8,000 meticulously crafted questions that test arithmetic, reasoning, and problem-solving skills. The benchmark measures both accuracy and the ability to follow multi-step reasoning processes to arrive at correct solutions.
-
MATH: The Mathematics Aptitude Test of Heuristics (MATH), Level 5, features challenging high school-level competition problems designed to test mathematical aptitude. The content covers a wide range of topics, including complex algebra, intricate geometry problems, and advanced calculus, offering a rigorous evaluation of problem-solving skills.
-
IFEVAL: Designed to evaluate the ability of language models to perform instruction following tasks across diverse domains, focusing on their alignment with human-provided instructions.
These benchmarks provide a comprehensive framework for evaluating LLMs, each focusing on distinct aspects of language understanding and generation.
The MMLU benchmark encompasses a diverse range of subjects, each assessed using carefully designed test datasets. For each subject, the LLM generates responses that are compared against a set of correct answers to evaluate its accuracy. The benchmark is designed to test the model's knowledge, reasoning, and understanding across multiple domains, including STEM, humanities, and social sciences. The final performance is computed by aggregating the accuracy scores across all subjects, providing a comprehensive measure of the model's capabilities in multitask language understanding.
Abstract Algebra | Astronomy | College Biology | College Chemistry |
---|---|---|---|
College Computer Science | College Mathematics | College Physics | Computer Security |
Conceptual Physics | Electrical Engineering | Elementary Mathematics | High School Biology |
High School Chemistry | High School Computer Science | High School Mathematics | High School Physics |
High School Statistics | Machine Learning | Jurisprudence | Philosophy |
World Religions | Econometrics | High School Economics | High School Geography |
High School Government and Politics | High School Macroeconomics | High School Microeconomics | High School Psychology |
International Law | Logical Fallacies | Prehistory | Professional Law |
Public Relations | Security Studies | Sociology | US Foreign Policy |
US History | World History | Business Ethics | Clinical Knowledge |
College Medicine | Global Facts | High School European History | High School World History |
Human Aging | Human Sexuality | Management | Marketing |
Medical Genetics | Nutrition | Professional Accounting | Professional Medicine |
Professional Psychology | Virology |
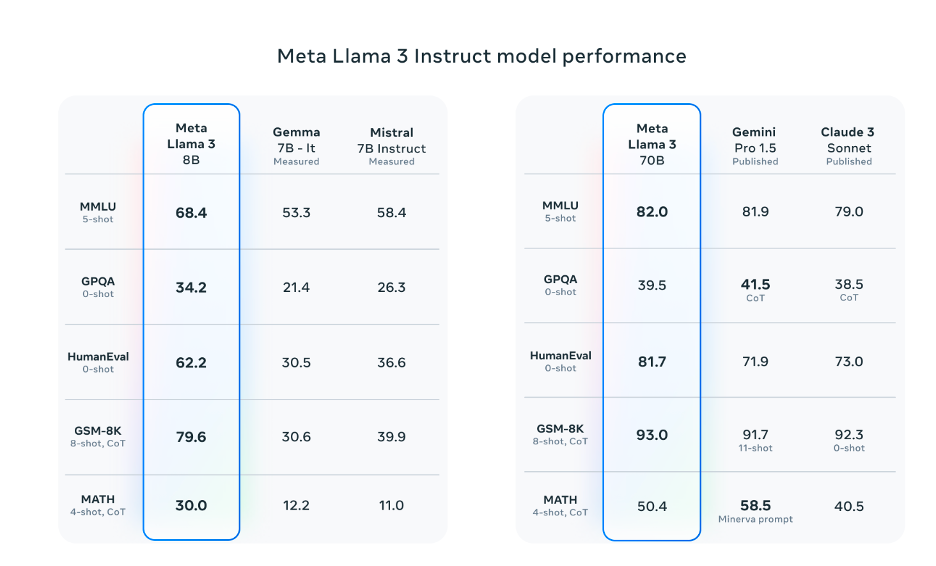
Fig: LLM model performance across different benchmarks
Reference:
https://ai.meta.com/blog/meta-llama-3/
https://build.nvidia.com/meta/llama-3_3-70b-instruct/modelcard
Conclusion:
The customization of LLMs is crucial for enhancing domain-specific accuracy, task performance, and operational efficiency, addressing unique business needs. Pre-training establishes a strong foundation, while fine-tuning aligns models with specific applications, making them more relevant and efficient. Benchmarks like MMLU and HumanEval ensure rigorous evaluation of LLM capabilities, driving continuous improvement. As LLMs evolve, their integration into scalable and efficient production environments will shape the future of AI, revolutionizing the way we interact with technology. By leveraging customization and robust evaluation frameworks, industries can unlock the full potential of LLMs for transformative impact.
Additional Reading: