Facilitating Dark DevOps with ChatOps and Agentic AI
INTRODUCTION
Advent of AI, IoT, Web 3.0, Quantum Computing, AR/VR has ushered in the era of 4th Industrial Revolution. As this 4IR world unveils itself, the boundary between the real and the digital world blurs. Humans prefer to interact with the real world using their virtual world and vice versa.
The factory in the 4IR is known as Dark Factory. These factories are massively automated using AI enabled robots. As no human beings are present on the floor, hence there is no requirement for lighting, and it is switched off. Thus, the name Dark Factory.
The field of Software Engineering is not untouched by 4IR concepts. A Modern Software development is facilitated by DevOps. We can consider DevOps as a factory which produces code as final output. Consequently, a true 4IR DevOps factory must be heavily automated using AI and the resultant is called Dark DevOps. The Generative AI and Agentic AI makes the setup of Dark DevOps even easier and makes it ripe for innovation.
Another trend which is shaping the DevOps is ChatOps. ChatOps is a methodology that consolidates team communication, task execution, and information sharing in a central chat platform. Imagine a team using multitude of tools across the SDLC lifecycle to perform their tasks. The team has to struggle to collaborate with different teams using many different tools.
In this blog post we will see how to improve efficiency of a DevOps/SDLC processes using concepts of ChatOps and Agentic AI.
PROBLEM DEFINITION
Before moving on let's have a discussion on what really troubles the most SDLC teams.
- Collaboration Overhead - Collaboration with multiple teams. Each in their own Silo resulting in lost messages and too much time spent on conveying similar information.
- Findability - Finding information from multiple sources which could be internal or external.
- TOIL - TOIL are the repetitive tasks which teams must perform. As per google survey they are the major sources of burnout for software engineer.
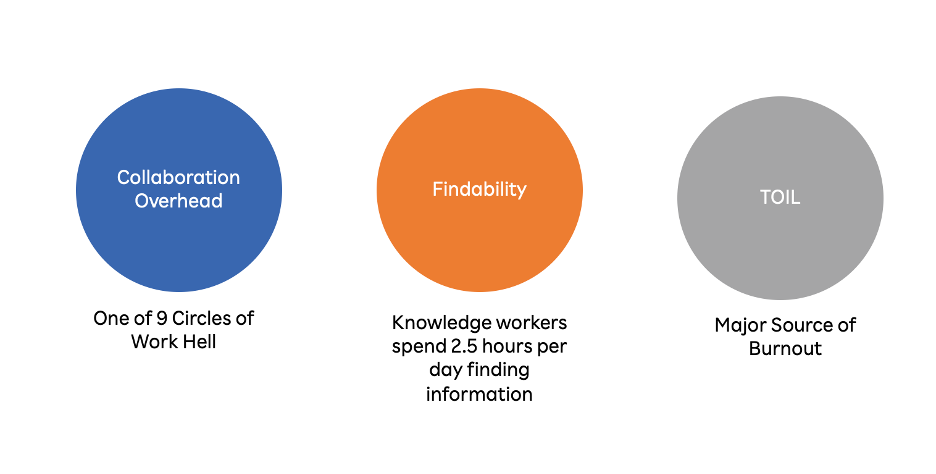
ENABLING TECHNOLOGIES
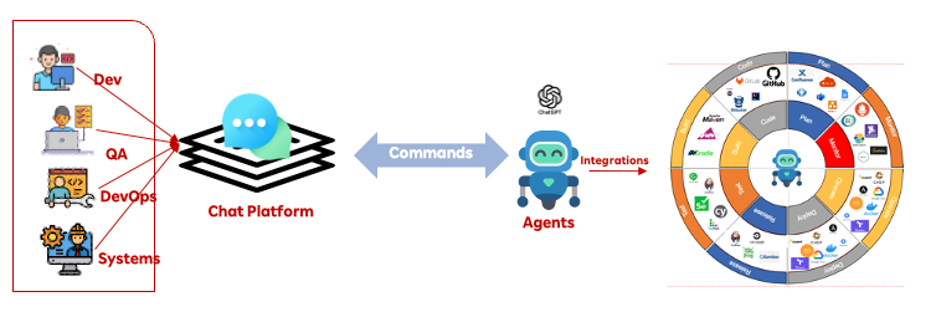
Figure 1: AI Enabled ChatOps Platform
The core components of AI Enabled ChatOps are as follows:
- Input Command: A user types a command or message in the chat platform. The input commands are converted to actual software command using NLP techniques as explained below.
- Chat Platform: which enables real-time communication and collaboration through text-based messaging like Slack, Teams etc.
- AI enabled Bot: The bot parses the command, determines the action to take, and interacts with the appropriate external system or API. It does all this using an intelligent AI engine which are read understand and parse the command.
- Action Execution: The bot executes the requested action, such as running a script, querying a database, or triggering a deployment.
- Feedback: The bot sends the results or status updates back to the chat, visible to the entire team.
- SDLC Tools: are software applications or platforms that help teams manage, automate, and streamline the various stages of the software development life cycle. For example, Confluence, Jira, SonarQube, TestRail, Cloud Native Tools, Alerting and monitoring tools etc.
USE CASES
Collaboration
SDLC team in an enterprise must communicate and collaborate with many other teams get their work done. This creates silos of information pockets and creates a large collaboration overhead for any team. There is also loss of important messages which may hurt the teams in terms of delivery.
For example, imagine a technical decision which is arrived at in an isolated chat between members of two teams. This decision must be shared with other team members and listed in Architecture Decision Record. Though there are chances that this discussion is never shared and finally lost.
With the Centralized Chat Platform of ChatOps and AI enabled bots to manage the communication, we can consolidate and share many of the important messages with the team members.
Findability
Finding Information from Internet
With Integration of Generative AI tools with the different DevOps agents, SDLC teams can send their general web-based search queries to agents and get a consolidated answer.
Finding Information from Knowledge tools like Jira, Confluence etc.
Project information is scattered in multiple SDLC tools like Confluence, JIRA etc. Team members may struggle to glean information from these tools.
Agentic AI integrated with tools like Confluence and Jira, using RAG frameworks can greatly enhance the team's efficiency in finding relevant project related information and share it on the chat itself.
Reporting
Again, Project managers and team member must pull SDLC reports from various tools. Jira for sprint reports, Test reports from some test management tools, Code Quality Reports from SonarQube, different Observability reports from Observability tools.
Agentic AI integrated with tools like Sonar, Jira, Prometheus, PagerDuty, TestRail etc. can easily retrieve reports from these tools and serve it on the centralized chat platform.
Custom Save and Retrieve Tool
Team can share important links along with some metadata about the link to Agentic AI. The AI can save and index it and serve the relevant links when required. Thus, teams can create a custom team bookmark tool over the Chat Platform.
TOIL Reduction
System Health Monitoring
Agentic AI integrated with a monitoring tool can easily pull the system health information like error rate, exceptions, component failure etc. For example, an agent integrated with Prometheus can easily generate PromQL from command send by user from chat. It can send the PromQL to Prometheus and get relevant system health information.
Kubernetes Integration
As more and more applications are deployed on Kubernetes, Agentic AI integrated with Kubernetes, can enable teams in doing common Kubernetes tasks, right from the Chat interface. They can look at pod states, YAML files, executing Kubernetes commands, logs etc., right from the chat.
Intelligent Log Analysis
Agents Kubernetes can pull the logs from different components. Furthermore, the AI can analyze and summarize the logs. This can help in RCA of critical events and faster resolution of production issue.
Alert Enrichment
A production incident normally results in an alert which is normally sent to emails, chats and phones. The chat-based alerts can be read automatically by the bot, and it can pull information from Kubernetes, Logs, monitoring systems to enrich the alerts and create a centralized system.
Enabling CaaS and IaaS and Lights-Out
CaaS and IaaS are normally managed by tools like chef, ansible, terraform etc. Once these tools are integrated with a Agentic AI they can perform various configuration and infrastructure tasks like scaling, failovers, configuration management, running Linux commands etc. right from the chat platform. This can also be converted to a lights-out system when AI can automatically detect and resolve issues with help of a human partner on the chat. Thus, creating a true Dark DevOps.
CHALLENGES AND ETHICAL CONSIDERATIONS
Use of Agentic AI integrated with SDLC tools over ChatOps may seems promising, but they are not free of risks and challenges.
- Data Risk and Compliance - Sending over alerts and logs and sensitive operational data to Open AI may pose security risk, thus proper anonymization is required to prevent data leaks and other security incidents.
- Hallucinations and False Positives - Agentic AI may incorrectly interpret the data and provide a false solution. Thus, we need a human in loop to verify and execute.
- Scale and Cost - Kubernetes and monitoring systems generate large amount of data, and it may result in huge API cost. Thus, care is required in limiting the data sent using rate limits.
- Audit - The AI actions should be logged and audited to reduce risk.
- Trust - We need explainable AI which explains the actions and recommendations to the DevOps and SRE teams. This fosters environment of trust over time.
CONCLUSION
We saw that Agentic AI integrated with various SDLC tools can make life of software engineers easy. No more juggling with multiple chat platforms and tools for day-to-day work. They can automate all the repetitive grunt work with help of AI. Though as cliché goes “with great power comes great responsibilities”. The engineers must be extra careful as provide proper audit-based access, anonymize the data shared with AI and ensure that cost of AI API is managed closely.