Rethinking AI Hallucinations: Unlocking New Possibilities
Introduction:
Artificial intelligence (AI) is rapidly transforming industries, and Large Language Models (LLMs) are at the forefront of this revolution. While LLMs have demonstrated impressive capabilities in tasks like text generation and translation, they also exhibit a phenomenon known as "hallucination," where they generate factually incorrect or nonsensical outputs.
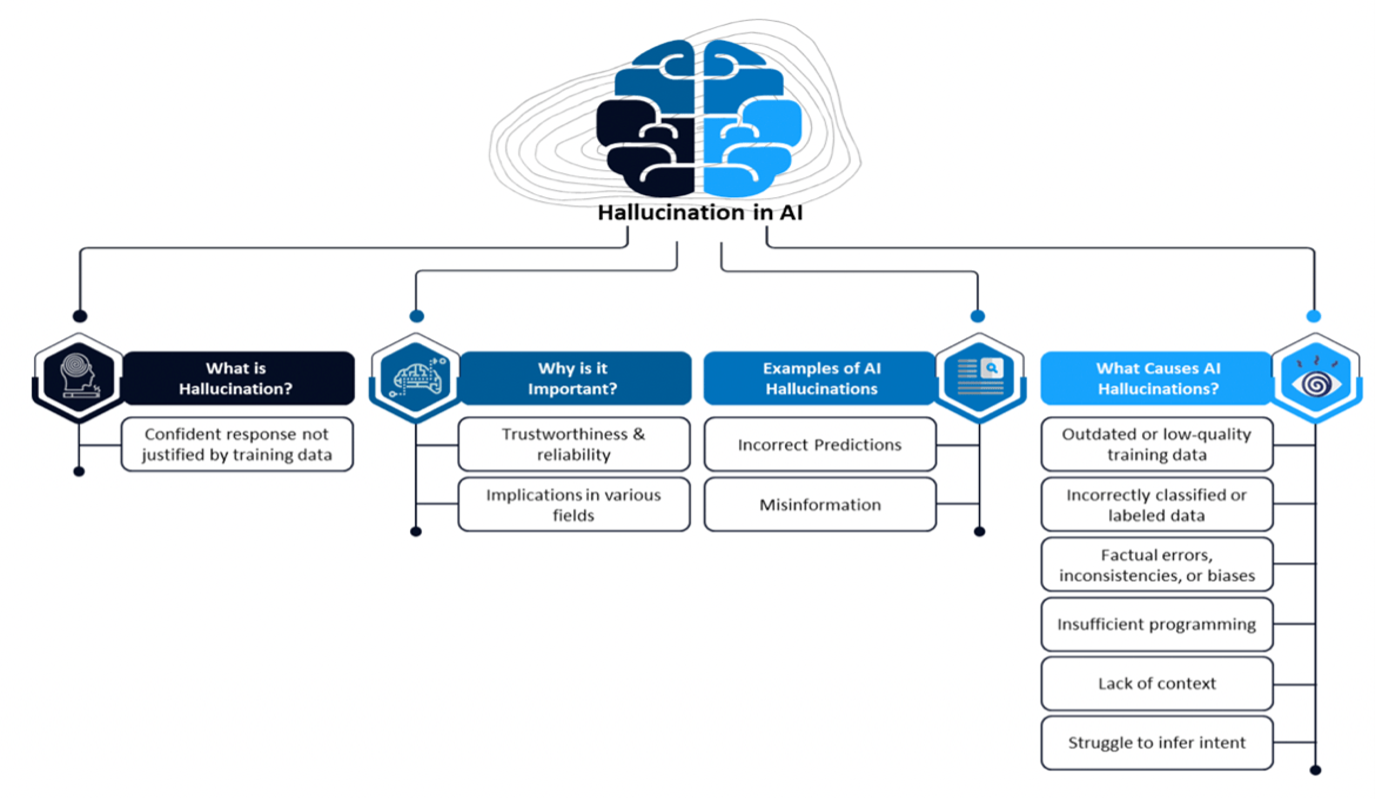
What if AI’s so-called “flaws” could be its greatest strength? The tendency of AI to "hallucinate"—to generate outputs beyond the given data—is often seen as a problem. But what if we could harness this trait to unlock new possibilities? In this blog, we explore how AI hallucinations can be leveraged to derive rich, imaginative context metadata from basic database schemas. You’ll discover how AI can transform raw technical metadata into meaningful business insights, automate metadata generation, and inspire innovative ways to maximize data utility. Whether it’s creating detailed descriptions for columns or uncovering new strategies to increase table utilization, this approach challenges conventional thinking and redefines how we interact with data. Read on to learn how embracing AI’s creative potential can drive smarter, more innovative data-driven decisions.
Artificial Intelligence (AI) is often celebrated for its precision, efficiency, and ability to process vast amounts of data. However, one of its most debated traits is its tendency to "hallucinate"—to generate outputs that are not grounded. This is often seen as a flaw, especially in scenarios where factual accuracy is critical.
For example, imagine asking an AI model to solve a simple math problem like 2 + 2, and it hallucinates the answer as 5. This is a clear failure because the result is objectively incorrect. Similarly, in fields like finance, medicine, or legal documentation, hallucination can lead to serious consequences.
While hallucination can be problematic in factual scenarios, it has proven to be a powerful tool for creativity and innovation in fields like drug discovery. Traditional drug discovery relies heavily on empirical data and known chemical structures, but breakthroughs often require exploring unconventional ideas. This is where AI’s ability to hallucinate shines.
For more on how hallucinations are driving innovation in AI, check out this article from Psychology Today: Harnessing Hallucinations to Make AI More Creative.
Problem Definition:
While technical and operational metadata are relatively straightforward to capture, generating context metadata—the descriptions and business context that explain the purpose and functionality of data—is a complex and time-consuming process. This is because data owners are often required to manually write context and descriptions for every individual table and column, which can be overwhelming in large systems. It is far easier for business analysts to review descriptions versus writing them from scratch. Additionally, in cases where the data provider is a system, such as a big data platform, there may not be a specific data owner available to provide these details. This lack of human input makes it challenging to derive accurate context and increases the risk of human error, leading to incomplete or inconsistent metadata. This is where AI hallucination becomes invaluable, automating the process and filling in the gaps with creative, plausible insights.
Enabling Technologies:
Recent advances in LLMs have made it possible to generate human-like text with increasing accuracy and fluency. By fine-tuning these models on datasets of database schemas and their corresponding descriptions, we can train them to "hallucinate" plausible and relevant descriptions for new schemas. The key lies in prompting engineering – crafting specific prompts that guide the LLM towards generating useful information. For instance, prompts can include keywords related to the industry, domain, or type of data expected in the table.
Use Cases:
Leveraging Hallucination in Rakuten: Deriving Context Metadat In Rakuten’s Metadata Management Service, we’ve embraced the creative potential of AI hallucinations to solve a critical problem in metadata management: generating context metadata. As part of Rakuten’s Metadata Management Service, we consolidate metadata from various data sources, including:
-
Technical Metadata: Information about tables, columns, data types, and schemas.
-
Operational Metadata: Details about data lineage, refresh frequency, and processing times.
-
Dimensional Metadata: Contextual information about dimensions like time, geography, or customer segments.
-
Governance Metadata: Policies, access controls, and compliance-related information.
-
Statistical Metadata: Metrics like data completeness, accuracy, and distribution.
-
Quality Metadata: Information about data quality issues, such as missing values or inconsistencies.
-
Business Context Metadata: Descriptions of how the data is used in business processes, including its purpose and relevance.
How Hallucination Helps in Rakuten
By analyzing the available metadata (technical, operational, dimensional, etc.), AI can infer and generate context metadata and descriptions for tables and columns. This process involves identifying patterns, relationships, and usage scenarios to generate meaningful insights.
Example: The <service>_usage Table
Let’s take the <service>_usage table as an example. This table contains information about customer activity on this service including order history and engagement metrics.
Technical Metadata (Input):
Table Name: <service>_usage
Columns:
<service>_order_recency_cd (INT)
first_<service>_order_dt (STRING)
last_<service>_order_dt (STRING)
l3m_<service>_order_count (INT)
AI derived description: AI can infer that a column like l3m_ <service> order_count (last 3 months' order count for a service) represents short-term customer engagement. This insight can be used to identify active customers and design targeted marketing campaigns, increasing customer retention and sales.
While AI can generate context metadata and provide valuable insights, human feedback loops are critical before approving the derived context metadata. Domain experts review and validate the AI-generated descriptions to ensure they align with the actual business use case and data purpose. This step is essential to avoid misinterpretation and ensure the metadata is accurate, relevant, and actionable.
By combining AI’s ability to hallucinate creative insights with human expertise, businesses can confidently leverage context metadata to unlock new opportunities, improve data utilization, and expand their operations.
How Derived Context Can Be a Breakthrough for Business Expansion
The same principle applies to metadata management. By leveraging hallucination to derive context metadata, businesses can unlock new ways to utilize their data and expand their operations. For example:
Derived business context can suggest combining internal data with external sources, such as demographic or weather data, to uncover new patterns. For instance, correlating order trends with weather conditions could lead to region-specific promotions, unlocking new revenue streams.
While AI hallucination is certainly an unwanted outcome in most cases, it also presents a range of intriguing use cases that can help organizations leverage its creative potential in positive ways. Some other interesting use cases are:
-
Art and design:
AI hallucination offers a novel approach to artistic creation, providing artists, designers and other creatives a tool for generating visually stunning and imaginative imagery. With the hallucinatory capabilities of artificial intelligence, artists can produce surreal and dream-like images that can generate new art forms and styles.
-
Data visualization and interpretation:
AI hallucination can streamline data visualization by exposing new connections and offering alternative perspectives on complex information. This can be particularly valuable in fields such as finance, where visualizing intricate market trends and financial data facilitates more nuanced decision-making and risk analysis.
-
Gaming and virtual reality (VR):
AI hallucination also enhances immersive experiences in gaming and VR. Employing AI models to hallucinate and generate virtual environments can help game developers and VR designers imagine new worlds that take the user experience to the next level. Hallucination can also add an element of surprise, unpredictability and novelty to gaming experiences.
Conclusion: Embracing AI Hallucination for Innovation
AI hallucination is often viewed as a flaw, but when applied thoughtfully, it can be a powerful tool for creativity and innovation. By leveraging hallucination to derive context metadata, Rakuten’s Metadata Management service transforms raw metadata into actionable business intelligence. But beyond its practical benefits, AI’s creative potential is what truly sets it apart. By "hallucinating" new ideas and possibilities, AI can push the boundaries of conventional thinking and inspire innovation. It challenges us to rethink how we interact with data, encouraging us to explore new opportunities and unlock hidden value.
As we continue to embrace AI in metadata management, it’s important to strike a balance between precision and imagination. While factual accuracy is critical, the ability to think beyond the data and explore new possibilities is equally valuable. After all, progress often begins where convention ends.
So, the next time you encounter an AI-generated insight that seems unconventional or unexpected, don’t dismiss it outright. Instead, ask yourself: What if this is the spark of a breakthrough?