Revolutionizing Retail: How LLMs Are Redefining Personalization
Introduction: The Evolution of Retail Commerce
Retail has always been about understanding customers—whether it was the local shopkeeper who knew everyone's preferences or today's e-commerce platforms that cater to millions of users. Over the years, retail has transformed from a transactional experience to a relationship-driven journey, where customers expect businesses to anticipate their needs and deliver tailored experiences.
In the digital age, personalization has become the cornerstone of retail success. From product recommendations to dynamic pricing, businesses are leveraging technology to create unique shopping experiences. But is personalization truly personal? Or are most systems still relying on generic clustering methods that fail to capture the nuances of individual preferences? This blog explores how Large Language Models (LLMs) are reshaping personalization in retail, unlocking new possibilities for businesses of all sizes.
The Growing Importance of Personalization in Retail
Why Personalization Matters
Personalization is no longer a luxury—it's a necessity. Customers expect businesses to understand their preferences and deliver experiences that feel relevant and meaningful. According to Epsilon, 80% of consumers are more likely to purchase from brands that offer personalized experiences, and 90% find personalization appealing. This highlights the growing demand for tailored interactions that make customers feel valued.
Moreover, McKinsey & Company reports that companies excelling at personalization generate 40% more revenue than their competitors. These statistics underscore the importance of personalization as a key driver of customer satisfaction, loyalty, and business growth.
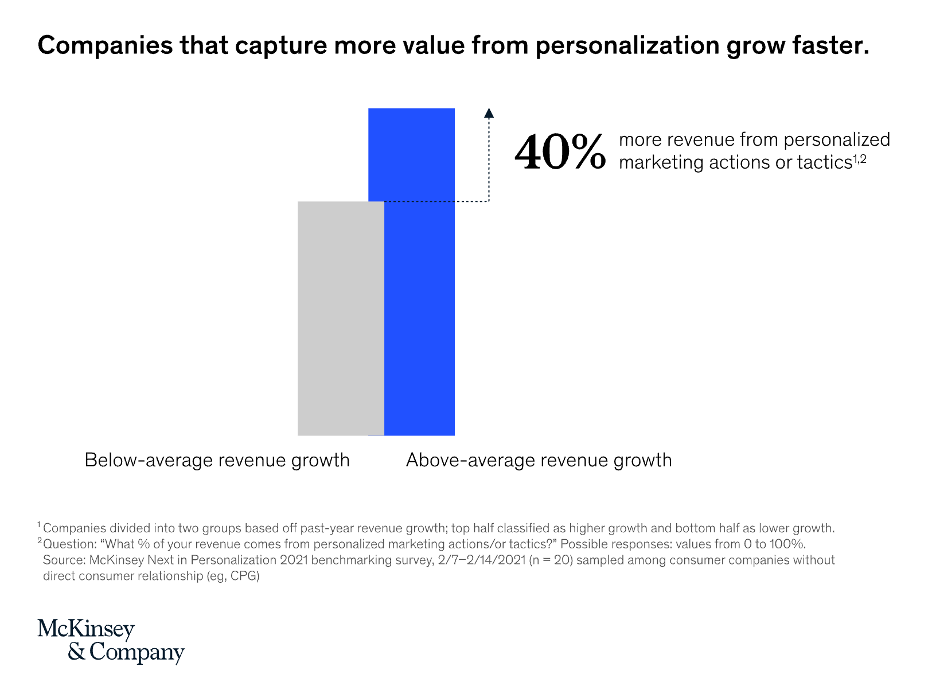
However, while personalization is widely adopted, many systems fall short of delivering truly individualized experiences. Here's why:
-
Clustering-Based Approaches:
Most personalization systems rely on clustering algorithms that group customers based on shared characteristics like age, location, or purchase history. While effective for broad segmentation, this approach often lacks the depth to understand individual preferences.
-
Sparse and Complex Data:
Personal data is often scattered across multiple platforms and formats, making it difficult to extract meaningful insights. For example, a customer's unique preferences might be buried in a mix of purchase history, reviews, and social media interactions.
-
Small Business Limitations:
Large retailers like Amazon have the resources to implement advanced AI systems, but smaller businesses often rely on off-the-shelf tools that provide basic personalization features.
How Retailers Are Approaching Personalization
Amazon's Advanced Personalization
Amazon sets the gold standard for personalization by using collaborative filtering and deep learning models to recommend products based on individual behavior and cross-customer patterns. For example:
-
Collaborative Filtering: Amazon analyzes the behavior of similar users to recommend products.
-
Deep Learning: Neural networks process vast amounts of data, including purchase history and browsing behavior, to generate personalized recommendations.
However, such advanced systems are out of reach for many smaller businesses, which often rely on simpler clustering-based methods. This is where Large Language Models (LLMs) come into play, offering a more accessible and effective approach to personalization.
How LLMs Are Transforming Personalization
Large Language Models (LLMs), such as GPT, are revolutionizing how businesses approach personalization. Unlike traditional systems, LLMs can process and generate human-like text, making them ideal for understanding and responding to customer needs.
Moving Beyond Clustering with LLMs
One of the key limitations of clustering-based personalization is its inability to capture the nuances of individual preferences. LLMs address this by analyzing unstructured data—such as customer reviews, chat logs, and social media posts—to create a more comprehensive understanding of each customer.
For example, instead of grouping customers into broad categories, LLMs can generate personalized profiles that highlight unique preferences. These profiles summarize key insights from a customer's data, such as their preferred product categories, shopping habits, and communication style. By using these profiles, businesses can tailor their recommendations, marketing messages, and customer interactions to align more closely with individual needs.
Enhancing Personalization with Contextual Profiles
One of the most powerful ways to improve personalization is by creating contextual profiles that summarize a customer's unique preferences in natural language. These profiles act as a bridge between raw data and actionable insights, enabling businesses to deliver more precise and relevant experiences.
How Contextual Profiles Work
-
Digesting Personal Context:
Instead of relying on raw data like purchase history or browsing behavior, contextual profiles extract the most important and distinctive features from a customer's data. For example, a profile might highlight that a customer frequently buys eco-friendly products or prefers casual communication styles.
-
Generating Descriptive Profiles:
These profiles are written in natural language, making them easy to interpret and use across different personalization tasks. For instance:
"This customer prefers eco-friendly products and frequently buys skincare items." -
Tailoring Outputs:
The profiles are then used to guide LLMs in generating personalized recommendations, chatbot responses, or marketing content. This ensures that every interaction feels relevant and meaningful to the customer.
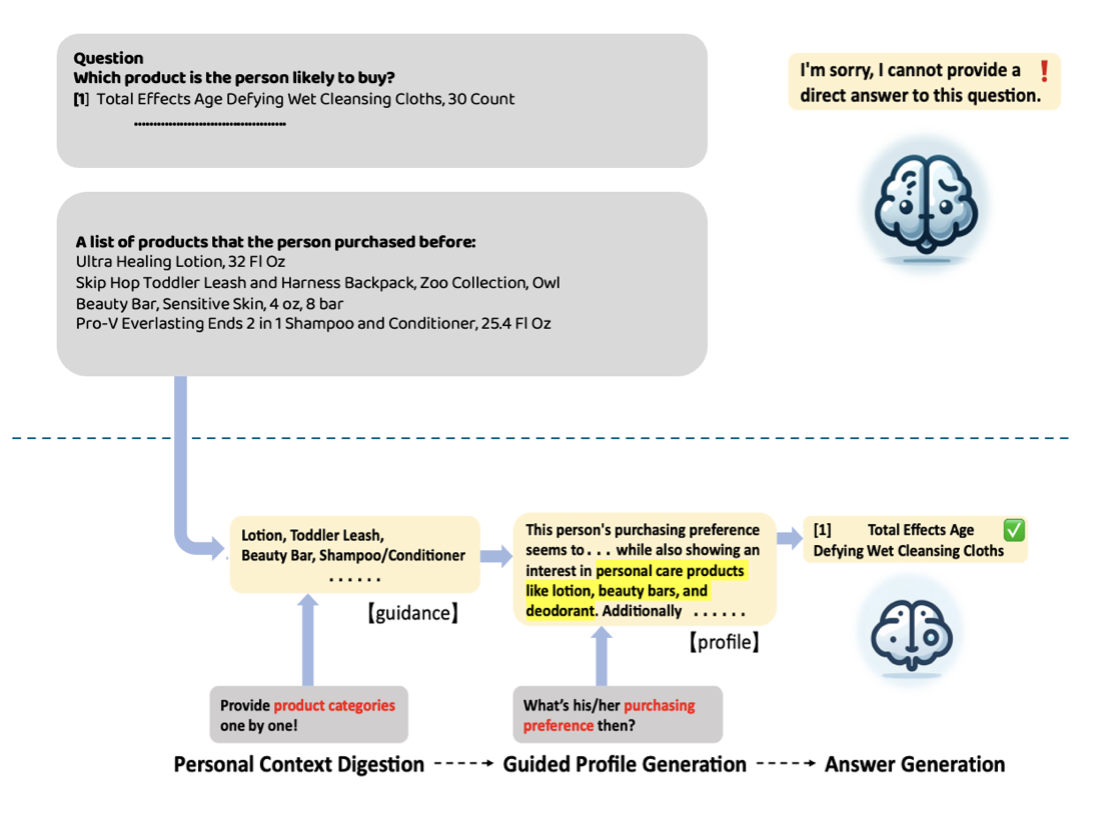
By leveraging contextual profiles, businesses can move beyond generic recommendations and deliver truly personalized experiences that resonate with their customers.
Innovative Applications of LLMs in Personalization in Retail
-
Hyper-Personalized Recommendations
LLMs can analyze a customer's entire interaction history—browsing behavior, purchase history, and even chat conversations—to provide recommendations tailored to their unique preferences.
-
Emotion-Aware Interactions
By analyzing sentiment in customer reviews or chat messages, LLMs can detect emotions and adjust their responses accordingly. For example:
-
A frustrated customer might receive empathetic support and a discount offer.
-
A happy customer might be upsold complementary products.
-
-
Personalized Product Descriptions
LLMs can generate product descriptions that highlight features relevant to a specific customer. For example:
-
A customer interested in eco-friendly products might see descriptions emphasizing sustainability.
-
A tech-savvy customer might see descriptions focusing on advanced features.
-
-
Conversational Commerce
LLMs enable conversational commerce, where customers can interact with a virtual assistant to discover products, ask questions, and complete purchases—all in a single conversation. Personalization enhances this experience by:
-
Tailoring Conversations: The virtual assistant can adapt its tone and recommendations based on the customer's preferences and past interactions. For example, a customer who frequently buys skincare products might receive personalized advice on new arrivals or complementary items.
-
Dynamic Recommendations: As the conversation progresses, the assistant can refine its suggestions based on the customer's responses, creating a more engaging and relevant shopping experience.
-
Future Prospects: The Next Frontier of Personalization
The future of personalization in retail is exciting, with several trends on the horizon:
-
Hyper-Personalization at Scale
Advancements in AI will enable businesses to deliver hyper-personalized experiences to millions of customers simultaneously.
-
Ethical Personalization
As concerns about data privacy grow, businesses will need to adopt transparent and ethical AI practices, giving customers more control over their data.
-
Multimodal Personalization
Future systems will integrate text, images, and even sensor data (e.g., heart rate or location) to create richer, more holistic personalization experiences.
Conclusion
Personalization is the key to success in retail, but it's no longer enough to rely on clustering-based approaches. With the rise of LLMs and the ability to generate contextual profiles, businesses can deliver truly personalized experiences that delight customers and drive growth. From hyper-personalized recommendations to conversational commerce, the possibilities are endless.
As we look to the future, the focus will shift toward hyper-personalization, emotion-aware AI, and ethical practices. By embracing these trends, businesses of all sizes can stay ahead in the ever-evolving world of retail.
References